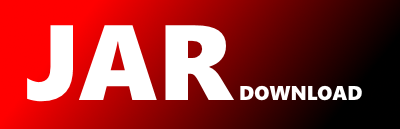
org.numenta.nupic.Connections Maven / Gradle / Ivy
Go to download
Show more of this group Show more artifacts with this name
Show all versions of htm.java Show documentation
Show all versions of htm.java Show documentation
The Java version of Numenta's HTM technology
/* ---------------------------------------------------------------------
* Numenta Platform for Intelligent Computing (NuPIC)
* Copyright (C) 2014, Numenta, Inc. Unless you have an agreement
* with Numenta, Inc., for a separate license for this software code, the
* following terms and conditions apply:
*
* This program is free software: you can redistribute it and/or modify
* it under the terms of the GNU General Public License version 3 as
* published by the Free Software Foundation.
*
* This program is distributed in the hope that it will be useful,
* but WITHOUT ANY WARRANTY; without even the implied warranty of
* MERCHANTABILITY or FITNESS FOR A PARTICULAR PURPOSE.
* See the GNU General Public License for more details.
*
* You should have received a copy of the GNU General Public License
* along with this program. If not, see http://www.gnu.org/licenses.
*
* http://numenta.org/licenses/
* ---------------------------------------------------------------------
*/
package org.numenta.nupic;
import java.util.ArrayList;
import java.util.Arrays;
import java.util.Collection;
import java.util.LinkedHashMap;
import java.util.LinkedHashSet;
import java.util.List;
import java.util.Map;
import java.util.Random;
import java.util.Set;
import org.numenta.nupic.model.Cell;
import org.numenta.nupic.model.Column;
import org.numenta.nupic.model.DistalDendrite;
import org.numenta.nupic.model.Pool;
import org.numenta.nupic.model.ProximalDendrite;
import org.numenta.nupic.model.Segment;
import org.numenta.nupic.model.Synapse;
import org.numenta.nupic.research.SpatialPooler;
import org.numenta.nupic.research.TemporalMemory;
import org.numenta.nupic.util.MersenneTwister;
import org.numenta.nupic.util.SparseBinaryMatrix;
import org.numenta.nupic.util.SparseMatrix;
import org.numenta.nupic.util.SparseObjectMatrix;
/**
* Contains the definition of the interconnected structural state of the {@link SpatialPooler} and
* {@link TemporalMemory} as well as the state of all support structures
* (i.e. Cells, Columns, Segments, Synapses etc.).
*
* In the separation of data from logic, this class represents the data/state.
*/
public class Connections {
/////////////////////////////////////// Spatial Pooler Vars ///////////////////////////////////////////
private int potentialRadius = 16;
private double potentialPct = 0.5;
private boolean globalInhibition = false;
private double localAreaDensity = -1.0;
private double numActiveColumnsPerInhArea;
private double stimulusThreshold = 0;
private double synPermInactiveDec = 0.01;
private double synPermActiveInc = 0.10;
private double synPermConnected = 0.10;
private double synPermBelowStimulusInc = synPermConnected / 10.0;
private double minPctOverlapDutyCycles = 0.001;
private double minPctActiveDutyCycles = 0.001;
private int dutyCyclePeriod = 1000;
private double maxBoost = 10.0;
private int spVerbosity = 0;
private int numInputs = 1; //product of input dimensions
private int numColumns = 1; //product of column dimensions
//Extra parameter settings
private double synPermMin = 0.0;
private double synPermMax = 1.0;
private double synPermTrimThreshold = synPermActiveInc / 2.0;
private int updatePeriod = 50;
private double initConnectedPct = 0.5;
//Internal state
private double version = 1.0;
public int iterationNum = 0;
public int iterationLearnNum = 0;
/** A matrix representing the shape of the input. */
protected SparseMatrix> inputMatrix;
/**
* Store the set of all inputs that are within each column's potential pool.
* 'potentialPools' is a matrix, whose rows represent cortical columns, and
* whose columns represent the input bits. if potentialPools[i][j] == 1,
* then input bit 'j' is in column 'i's potential pool. A column can only be
* connected to inputs in its potential pool. The indices refer to a
* flattened version of both the inputs and columns. Namely, irrespective
* of the topology of the inputs and columns, they are treated as being a
* one dimensional array. Since a column is typically connected to only a
* subset of the inputs, many of the entries in the matrix are 0. Therefore
* the potentialPool matrix is stored using the SparseObjectMatrix
* class, to reduce memory footprint and computation time of algorithms that
* require iterating over the data structure.
*/
private SparseObjectMatrix potentialPools;
/**
* Initialize a tiny random tie breaker. This is used to determine winning
* columns where the overlaps are identical.
*/
private double[] tieBreaker;
/**
* Stores the number of connected synapses for each column. This is simply
* a sum of each row of 'connectedSynapses'. again, while this
* information is readily available from 'connectedSynapses', it is
* stored separately for efficiency purposes.
*/
private SparseBinaryMatrix connectedCounts;
/**
* The inhibition radius determines the size of a column's local
* neighborhood. of a column. A cortical column must overcome the overlap
* score of columns in its neighborhood in order to become actives. This
* radius is updated every learning round. It grows and shrinks with the
* average number of connected synapses per column.
*/
private int inhibitionRadius = 0;
private int proximalSynapseCounter = 0;
private double[] overlapDutyCycles;
private double[] activeDutyCycles;
private double[] minOverlapDutyCycles;
private double[] minActiveDutyCycles;
private double[] boostFactors;
/////////////////////////////////////// Temporal Memory Vars ///////////////////////////////////////////
protected Set activeCells = new LinkedHashSet();
protected Set winnerCells = new LinkedHashSet();
protected Set predictiveCells = new LinkedHashSet();
protected Set successfullyPredictedColumns = new LinkedHashSet();
protected Set activeSegments = new LinkedHashSet();
protected Set learningSegments = new LinkedHashSet();
protected Map> activeSynapsesForSegment = new LinkedHashMap>();
/** Total number of columns */
protected int[] columnDimensions = new int[] { 2048 };
/** Total number of cells per column */
protected int cellsPerColumn = 32;
/** What will comprise the Layer input. Input (i.e. from encoder) */
protected int[] inputDimensions = new int[] { 32, 32 };
/**
* If the number of active connected synapses on a segment
* is at least this threshold, the segment is said to be active.
*/
private int activationThreshold = 13;
/**
* Radius around cell from which it can
* sample to form distal {@link DistalDendrite} connections.
*/
private int learningRadius = 2048;
/**
* If the number of synapses active on a segment is at least this
* threshold, it is selected as the best matching
* cell in a bursting column.
*/
private int minThreshold = 10;
/** The maximum number of synapses added to a segment during learning. */
private int maxNewSynapseCount = 20;
/** Initial permanence of a new synapse */
private double initialPermanence = 0.21;
/**
* If the permanence value for a synapse
* is greater than this value, it is said
* to be connected.
*/
private double connectedPermanence = 0.50;
/**
* Amount by which permanences of synapses
* are incremented during learning.
*/
private double permanenceIncrement = 0.10;
/**
* Amount by which permanences of synapses
* are decremented during learning.
*/
private double permanenceDecrement = 0.10;
/** The main data structure containing columns, cells, and synapses */
private SparseObjectMatrix memory;
private Cell[] cells;
/////////////////////// Structural Elements /////////////////////////
/** Reverse mapping from source cell to {@link Synapse} */
protected Map> receptorSynapses;
protected Map> segments;
protected Map> synapses;
/** Helps index each new Segment */
protected int segmentCounter = 0;
/** Helps index each new Synapse */
protected int synapseCounter = 0;
/** The default random number seed */
protected int seed = 42;
/** The random number generator */
protected Random random = new MersenneTwister(42);
/**
* Constructs a new {@code Connections} object. Use
*
*/
public Connections() {}
/**
* Returns the configured initial connected percent.
* @return
*/
public double getInitConnectedPct() {
return this.initConnectedPct;
}
/**
* Clears all state.
*/
public void clear() {
activeCells.clear();
winnerCells.clear();
predictiveCells.clear();
successfullyPredictedColumns.clear();
activeSegments.clear();
learningSegments.clear();
activeSynapsesForSegment.clear();
}
/**
* Returns the segment counter
* @return
*/
public int getSegmentCount() {
return segmentCounter;
}
/**
* Sets the segment counter
* @param counter
*/
public void setSegmentCount(int counter) {
this.segmentCounter = counter;
}
/**
* Returns the cycle count.
* @return
*/
public int getIterationNum() {
return iterationNum;
}
/**
* Sets the iteration count.
* @param num
*/
public void setIterationNum(int num) {
this.iterationNum = num;
}
/**
* Returns the period count which is the number of cycles
* between meta information updates.
* @return
*/
public int getUpdatePeriod() {
return updatePeriod;
}
/**
* Sets the update period
* @param period
*/
public void setUpdatePeriod(int period) {
this.updatePeriod = period;
}
/**
* Returns the {@link Cell} specified by the index passed in.
* @param index of the specified cell to return.
* @return
*/
public Cell getCell(int index) {
return cells[index];
}
/**
* Returns an array containing all of the {@link Cell}s.
* @return
*/
public Cell[] getCells() {
return cells;
}
/**
* Sets the flat array of cells
* @param cells
*/
public void setCells(Cell[] cells) {
this.cells = cells;
}
/**
* Returns an array containing the {@link Cell}s specified
* by the passed in indexes.
*
* @param cellIndexes indexes of the Cells to return
* @return
*/
public Cell[] getCells(int[] cellIndexes) {
Cell[] retVal = new Cell[cellIndexes.length];
for(int i = 0;i < cellIndexes.length;i++) {
retVal[i] = cells[cellIndexes[i]];
}
return retVal;
}
/**
* Returns a {@link LinkedHashSet} containing the {@link Cell}s specified
* by the passed in indexes.
*
* @param cellIndexes indexes of the Cells to return
* @return
*/
public LinkedHashSet getCellSet(int[] cellIndexes) {
LinkedHashSet retVal = new LinkedHashSet(cellIndexes.length);
for(int i = 0;i < cellIndexes.length;i++) {
retVal.add(cells[cellIndexes[i]]);
}
return retVal;
}
/**
* Sets the seed used for the internal random number generator.
* If the generator has been instantiated, this method will initialize
* a new random generator with the specified seed.
*
* @param seed
*/
public void setSeed(int seed) {
this.seed = seed;
}
/**
* Returns the configured random number seed
* @return
*/
public int getSeed() {
return seed;
}
/**
* Returns the thread specific {@link Random} number generator.
* @return
*/
public Random getRandom() {
return random;
}
/**
* Sets the random number generator.
* @param random
*/
public void setRandom(Random random){
this.random = random;
}
/**
* Sets the matrix containing the {@link Column}s
* @param mem
*/
public void setMemory(SparseObjectMatrix mem) {
this.memory = mem;
}
/**
* Returns the matrix containing the {@link Column}s
* @return
*/
public SparseObjectMatrix getMemory() {
return memory;
}
/**
* Returns the input column mapping
*/
public SparseMatrix> getInputMatrix() {
return inputMatrix;
}
/**
* Sets the input column mapping matrix
* @param matrix
*/
public void setInputMatrix(SparseMatrix> matrix) {
this.inputMatrix = matrix;
}
/**
* Returns the inhibition radius
* @return
*/
public int getInhibitionRadius() {
return inhibitionRadius;
}
/**
* Sets the inhibition radius
* @param radius
*/
public void setInhibitionRadius(int radius) {
this.inhibitionRadius = radius;
}
/**
* Returns the product of the input dimensions
* @return the product of the input dimensions
*/
public int getNumInputs() {
return numInputs;
}
/**
* Sets the product of the input dimensions to
* establish a flat count of bits in the input field.
* @param n
*/
public void setNumInputs(int n) {
this.numInputs = n;
}
/**
* Returns the product of the column dimensions
* @return the product of the column dimensions
*/
public int getNumColumns() {
return numColumns;
}
/**
* Sets the product of the column dimensions to be
* the column count.
* @param n
*/
public void setNumColumns(int n) {
this.numColumns = n;
}
/**
* This parameter determines the extent of the input
* that each column can potentially be connected to.
* This can be thought of as the input bits that
* are visible to each column, or a 'receptiveField' of
* the field of vision. A large enough value will result
* in 'global coverage', meaning that each column
* can potentially be connected to every input bit. This
* parameter defines a square (or hyper square) area: a
* column will have a max square potential pool with
* sides of length 2 * potentialRadius + 1.
*
* @param potentialRadius
*/
public void setPotentialRadius(int potentialRadius) {
this.potentialRadius = potentialRadius;
}
/**
* Returns the configured potential radius
* @return the configured potential radius
* @see {@link #setPotentialRadius(int)}
*/
public int getPotentialRadius() {
return Math.min(numInputs, potentialRadius);
}
/**
* The percent of the inputs, within a column's
* potential radius, that a column can be connected to.
* If set to 1, the column will be connected to every
* input within its potential radius. This parameter is
* used to give each column a unique potential pool when
* a large potentialRadius causes overlap between the
* columns. At initialization time we choose
* ((2*potentialRadius + 1)^(# inputDimensions) *
* potentialPct) input bits to comprise the column's
* potential pool.
*
* @param potentialPct
*/
public void setPotentialPct(double potentialPct) {
this.potentialPct = potentialPct;
}
/**
* Returns the configured potential pct
*
* @return the configured potential pct
* @see {@link #setPotentialPct(double)}
*/
public double getPotentialPct() {
return potentialPct;
}
/**
* Sets the {@link SparseObjectMatrix} which represents the
* proximal dendrite permanence values.
*
* @param s the {@link SparseObjectMatrix}
*/
public void setPermanences(SparseObjectMatrix s) {
for(int idx : s.getSparseIndices()) {
memory.getObject(idx).setProximalPermanences(
this, s.getObject(idx));
}
}
/**
* Returns the count of {@link Synapse}s
* @return
*/
public int getSynapseCount() {
return synapseCounter;
}
/**
* Sets the count of {@link Synapse}s
* @param i
*/
public void setSynapseCount(int i) {
this.synapseCounter = i;
}
/**
* Returns the indexed count of connected synapses per column.
* @return
*/
public SparseBinaryMatrix getConnectedCounts() {
return connectedCounts;
}
/**
* Returns the connected count for the specified column.
* @param columnIndex
* @return
*/
public int getConnectedCount(int columnIndex) {
return connectedCounts.getTrueCount(columnIndex);
}
/**
* Sets the indexed count of synapses connected at the columns in each index.
* @param counts
*/
public void setConnectedCounts(int[] counts) {
for(int i = 0;i < counts.length;i++) {
connectedCounts.setTrueCount(i, counts[i]);
}
}
/**
* Sets the connected count {@link SparseBinaryMatrix}
* @param columnIndex
* @param count
*/
public void setConnectedMatrix(SparseBinaryMatrix matrix) {
this.connectedCounts = matrix;
}
/**
* Sets the array holding the random noise added to proximal dendrite overlaps.
*
* @param tieBreaker random values to help break ties
*/
public void setTieBreaker(double[] tieBreaker) {
this.tieBreaker = tieBreaker;
}
/**
* Returns the array holding random values used to add to overlap scores
* to break ties.
*
* @return
*/
public double[] getTieBreaker() {
return tieBreaker;
}
/**
* If true, then during inhibition phase the winning
* columns are selected as the most active columns from
* the region as a whole. Otherwise, the winning columns
* are selected with respect to their local
* neighborhoods. Using global inhibition boosts
* performance x60.
*
* @param globalInhibition
*/
public void setGlobalInhibition(boolean globalInhibition) {
this.globalInhibition = globalInhibition;
}
/**
* Returns the configured global inhibition flag
* @return the configured global inhibition flag
* @see {@link #setGlobalInhibition(boolean)}
*/
public boolean getGlobalInhibition() {
return globalInhibition;
}
/**
* The desired density of active columns within a local
* inhibition area (the size of which is set by the
* internally calculated inhibitionRadius, which is in
* turn determined from the average size of the
* connected potential pools of all columns). The
* inhibition logic will insure that at most N columns
* remain ON within a local inhibition area, where N =
* localAreaDensity * (total number of columns in
* inhibition area).
*
* @param localAreaDensity
*/
public void setLocalAreaDensity(double localAreaDensity) {
this.localAreaDensity = localAreaDensity;
}
/**
* Returns the configured local area density
* @return the configured local area density
* @see {@link #setLocalAreaDensity(double)}
*/
public double getLocalAreaDensity() {
return localAreaDensity;
}
/**
* An alternate way to control the density of the active
* columns. If numActivePerInhArea is specified then
* localAreaDensity must be less than 0, and vice versa.
* When using numActivePerInhArea, the inhibition logic
* will insure that at most 'numActivePerInhArea'
* columns remain ON within a local inhibition area (the
* size of which is set by the internally calculated
* inhibitionRadius, which is in turn determined from
* the average size of the connected receptive fields of
* all columns). When using this method, as columns
* learn and grow their effective receptive fields, the
* inhibitionRadius will grow, and hence the net density
* of the active columns will *decrease*. This is in
* contrast to the localAreaDensity method, which keeps
* the density of active columns the same regardless of
* the size of their receptive fields.
*
* @param numActiveColumnsPerInhArea
*/
public void setNumActiveColumnsPerInhArea(double numActiveColumnsPerInhArea) {
this.numActiveColumnsPerInhArea = numActiveColumnsPerInhArea;
}
/**
* Returns the configured number of active columns per
* inhibition area.
* @return the configured number of active columns per
* inhibition area.
* @see {@link #setNumActiveColumnsPerInhArea(double)}
*/
public double getNumActiveColumnsPerInhArea() {
return numActiveColumnsPerInhArea;
}
/**
* This is a number specifying the minimum number of
* synapses that must be on in order for a columns to
* turn ON. The purpose of this is to prevent noise
* input from activating columns. Specified as a percent
* of a fully grown synapse.
*
* @param stimulusThreshold
*/
public void setStimulusThreshold(double stimulusThreshold) {
this.stimulusThreshold = stimulusThreshold;
}
/**
* Returns the stimulus threshold
* @return the stimulus threshold
* @see {@link #setStimulusThreshold(double)}
*/
public double getStimulusThreshold() {
return stimulusThreshold;
}
/**
* The amount by which an inactive synapse is
* decremented in each round. Specified as a percent of
* a fully grown synapse.
*
* @param synPermInactiveDec
*/
public void setSynPermInactiveDec(double synPermInactiveDec) {
this.synPermInactiveDec = synPermInactiveDec;
}
/**
* Returns the synaptic permanence inactive decrement.
* @return the synaptic permanence inactive decrement.
* @see {@link #setSynPermInactiveDec(double)}
*/
public double getSynPermInactiveDec() {
return synPermInactiveDec;
}
/**
* The amount by which an active synapse is incremented
* in each round. Specified as a percent of a
* fully grown synapse.
*
* @param synPermActiveInc
*/
public void setSynPermActiveInc(double synPermActiveInc) {
this.synPermActiveInc = synPermActiveInc;
}
/**
* Returns the configured active permanence increment
* @return the configured active permanence increment
* @see {@link #setSynPermActiveInc(double)}
*/
public double getSynPermActiveInc() {
return synPermActiveInc;
}
/**
* The default connected threshold. Any synapse whose
* permanence value is above the connected threshold is
* a "connected synapse", meaning it can contribute to
* the cell's firing.
*
* @param minPctOverlapDutyCycle
*/
public void setSynPermConnected(double synPermConnected) {
this.synPermConnected = synPermConnected;
}
/**
* Returns the synapse permanence connected threshold
* @return the synapse permanence connected threshold
* @see {@link #setSynPermConnected(double)}
*/
public double getSynPermConnected() {
return synPermConnected;
}
/**
* Sets the stimulus increment for synapse permanences below
* the measured threshold.
* @param stim
*/
public void setSynPermBelowStimulusInc(double stim) {
this.synPermBelowStimulusInc = stim;
}
/**
* Returns the stimulus increment for synapse permanences below
* the measured threshold.
*
* @return
*/
public double getSynPermBelowStimulusInc() {
return synPermBelowStimulusInc;
}
/**
* A number between 0 and 1.0, used to set a floor on
* how often a column should have at least
* stimulusThreshold active inputs. Periodically, each
* column looks at the overlap duty cycle of
* all other columns within its inhibition radius and
* sets its own internal minimal acceptable duty cycle
* to: minPctDutyCycleBeforeInh * max(other columns'
* duty cycles).
* On each iteration, any column whose overlap duty
* cycle falls below this computed value will get
* all of its permanence values boosted up by
* synPermActiveInc. Raising all permanences in response
* to a sub-par duty cycle before inhibition allows a
* cell to search for new inputs when either its
* previously learned inputs are no longer ever active,
* or when the vast majority of them have been
* "hijacked" by other columns.
*
* @param minPctOverlapDutyCycle
*/
public void setMinPctOverlapDutyCycles(double minPctOverlapDutyCycle) {
this.minPctOverlapDutyCycles = minPctOverlapDutyCycle;
}
/**
* {@see #setMinPctOverlapDutyCycles(double)}
* @return
*/
public double getMinPctOverlapDutyCycles() {
return minPctOverlapDutyCycles;
}
/**
* A number between 0 and 1.0, used to set a floor on
* how often a column should be activate.
* Periodically, each column looks at the activity duty
* cycle of all other columns within its inhibition
* radius and sets its own internal minimal acceptable
* duty cycle to:
* minPctDutyCycleAfterInh *
* max(other columns' duty cycles).
* On each iteration, any column whose duty cycle after
* inhibition falls below this computed value will get
* its internal boost factor increased.
*
* @param minPctActiveDutyCycle
*/
public void setMinPctActiveDutyCycles(double minPctActiveDutyCycle) {
this.minPctActiveDutyCycles = minPctActiveDutyCycle;
}
/**
* Returns the minPctActiveDutyCycle
* @return the minPctActiveDutyCycle
* @see {@link #setMinPctActiveDutyCycle(double)}
*/
public double getMinPctActiveDutyCycles() {
return minPctActiveDutyCycles;
}
/**
* The period used to calculate duty cycles. Higher
* values make it take longer to respond to changes in
* boost or synPerConnectedCell. Shorter values make it
* more unstable and likely to oscillate.
*
* @param dutyCyclePeriod
*/
public void setDutyCyclePeriod(int dutyCyclePeriod) {
this.dutyCyclePeriod = dutyCyclePeriod;
}
/**
* Returns the configured duty cycle period
* @return the configured duty cycle period
* @see {@link #setDutyCyclePeriod(double)}
*/
public int getDutyCyclePeriod() {
return dutyCyclePeriod;
}
/**
* The maximum overlap boost factor. Each column's
* overlap gets multiplied by a boost factor
* before it gets considered for inhibition.
* The actual boost factor for a column is number
* between 1.0 and maxBoost. A boost factor of 1.0 is
* used if the duty cycle is >= minOverlapDutyCycle,
* maxBoost is used if the duty cycle is 0, and any duty
* cycle in between is linearly extrapolated from these
* 2 end points.
*
* @param maxBoost
*/
public void setMaxBoost(double maxBoost) {
this.maxBoost = maxBoost;
}
/**
* Returns the max boost
* @return the max boost
* @see {@link #setMaxBoost(double)}
*/
public double getMaxBoost() {
return maxBoost;
}
/**
* spVerbosity level: 0, 1, 2, or 3
*
* @param spVerbosity
*/
public void setSpVerbosity(int spVerbosity) {
this.spVerbosity = spVerbosity;
}
/**
* Returns the verbosity setting.
* @return the verbosity setting.
* @see {@link #setSpVerbosity(int)}
*/
public int getSpVerbosity() {
return spVerbosity;
}
/**
* Sets the synPermTrimThreshold
* @param threshold
*/
public void setSynPermTrimThreshold(double threshold) {
this.synPermTrimThreshold = threshold;
}
/**
* Returns the synPermTrimThreshold
* @return
*/
public double getSynPermTrimThreshold() {
return synPermTrimThreshold;
}
/**
* Sets the {@link SparseObjectMatrix} which holds the mapping
* of column indexes to their lists of potential inputs.
*
* @param pools {@link SparseObjectMatrix} which holds the pools.
*/
public void setPotentialPools(SparseObjectMatrix pools) {
this.potentialPools = pools;
}
/**
* Returns the {@link SparseObjectMatrix} which holds the mapping
* of column indexes to their lists of potential inputs.
* @return the potential pools
*/
public SparseObjectMatrix getPotentialPools() {
return this.potentialPools;
}
/**
* Returns the minimum {@link Synapse} permanence.
* @return
*/
public double getSynPermMin() {
return synPermMin;
}
/**
* Returns the maximum {@link Synapse} permanence.
* @return
*/
public double getSynPermMax() {
return synPermMax;
}
/**
* Returns the output setting for verbosity
* @return
*/
public int getVerbosity() {
return spVerbosity;
}
/**
* Returns the version number
* @return
*/
public double getVersion() {
return version;
}
/**
* Returns the overlap duty cycles.
* @return
*/
public double[] getOverlapDutyCycles() {
return overlapDutyCycles;
}
public void setOverlapDutyCycles(double[] overlapDutyCycles) {
this.overlapDutyCycles = overlapDutyCycles;
}
/**
* Returns the dense (size=numColumns) array of duty cycle stats.
* @return the dense array of active duty cycle values.
*/
public double[] getActiveDutyCycles() {
return activeDutyCycles;
}
/**
* Sets the dense (size=numColumns) array of duty cycle stats.
* @param activeDutyCycles
*/
public void setActiveDutyCycles(double[] activeDutyCycles) {
this.activeDutyCycles = activeDutyCycles;
}
/**
* Applies the dense array values which aren't -1 to the array containing
* the active duty cycles of the column corresponding to the index specified.
* The length of the specified array must be as long as the configured number
* of columns of this {@code Connections}' column configuration.
*
* @param denseActiveDutyCycles a dense array containing values to set.
*/
public void updateActiveDutyCycles(double[] denseActiveDutyCycles) {
for(int i = 0;i < denseActiveDutyCycles.length;i++) {
if(denseActiveDutyCycles[i] != -1) {
activeDutyCycles[i] = denseActiveDutyCycles[i];
}
}
}
public double[] getMinOverlapDutyCycles() {
return minOverlapDutyCycles;
}
public void setMinOverlapDutyCycles(double[] minOverlapDutyCycles) {
this.minOverlapDutyCycles = minOverlapDutyCycles;
}
public double[] getMinActiveDutyCycles() {
return minActiveDutyCycles;
}
public void setMinActiveDutyCycles(double[] minActiveDutyCycles) {
this.minActiveDutyCycles = minActiveDutyCycles;
}
public double[] getBoostFactors() {
return boostFactors;
}
public void setBoostFactors(double[] boostFactors) {
this.boostFactors = boostFactors;
}
/**
* Returns the current count of {@link Synapse}s for {@link ProximalDendrite}s.
* @return
*/
public int getProxSynCount() {
return proximalSynapseCounter;
}
/**
* High verbose output useful for debugging
*/
public void printParameters() {
System.out.println("------------ SpatialPooler Parameters ------------------");
System.out.println("numInputs = " + getNumInputs());
System.out.println("numColumns = " + getNumColumns());
System.out.println("cellsPerColumn = " + getCellsPerColumn());
System.out.println("columnDimensions = " + Arrays.toString(getColumnDimensions()));
System.out.println("numActiveColumnsPerInhArea = " + getNumActiveColumnsPerInhArea());
System.out.println("potentialPct = " + getPotentialPct());
System.out.println("potentialRadius = " + getPotentialRadius());
System.out.println("globalInhibition = " + getGlobalInhibition());
System.out.println("localAreaDensity = " + getLocalAreaDensity());
System.out.println("inhibitionRadius = " + getInhibitionRadius());
System.out.println("stimulusThreshold = " + getStimulusThreshold());
System.out.println("synPermActiveInc = " + getSynPermActiveInc());
System.out.println("synPermInactiveDec = " + getSynPermInactiveDec());
System.out.println("synPermConnected = " + getSynPermConnected());
System.out.println("minPctOverlapDutyCycle = " + getMinPctOverlapDutyCycles());
System.out.println("minPctActiveDutyCycle = " + getMinPctActiveDutyCycles());
System.out.println("dutyCyclePeriod = " + getDutyCyclePeriod());
System.out.println("maxBoost = " + getMaxBoost());
System.out.println("spVerbosity = " + getSpVerbosity());
System.out.println("version = " + getVersion());
System.out.println("\n------------ TemporalMemory Parameters ------------------");
System.out.println("activationThreshold = " + getActivationThreshold());
System.out.println("learningRadius = " + getLearningRadius());
System.out.println("minThreshold = " + getMinThreshold());
System.out.println("maxNewSynapseCount = " + getMaxNewSynapseCount());
System.out.println("initialPermanence = " + getInitialPermanence());
System.out.println("connectedPermanence = " + getConnectedPermanence());
System.out.println("permanenceIncrement = " + getPermanenceIncrement());
System.out.println("permanenceDecrement = " + getPermanenceDecrement());
}
/////////////////////////////// Temporal Memory //////////////////////////////
/**
* Returns the current {@link Set} of active {@link Cell}s
*
* @return the current {@link Set} of active {@link Cell}s
*/
public Set getActiveCells() {
return activeCells;
}
/**
* Sets the current {@link Set} of active {@link Cell}s
* @param cells
*/
public void setActiveCells(Set cells) {
this.activeCells = cells;
}
/**
* Returns the current {@link Set} of winner cells
*
* @return the current {@link Set} of winner cells
*/
public Set getWinnerCells() {
return winnerCells;
}
/**
* Sets the current {@link Set} of winner {@link Cells}s
* @param cells
*/
public void setWinnerCells(Set cells) {
this.winnerCells = cells;
}
/**
* Returns the {@link Set} of predictive cells.
* @return
*/
public Set getPredictiveCells() {
return predictiveCells;
}
/**
* Sets the current {@link Set} of predictive {@link Cell}s
* @param cells
*/
public void setPredictiveCells(Set cells) {
this.predictiveCells = cells;
}
/**
* Returns the {@link Set} of columns successfully predicted from t - 1.
*
* @return the current {@link Set} of predicted columns
*/
public Set getSuccessfullyPredictedColumns() {
return successfullyPredictedColumns;
}
/**
* Sets the {@link Set} of columns successfully predicted from t - 1.
* @param columns
*/
public void setSuccessfullyPredictedColumns(Set columns) {
this.successfullyPredictedColumns = columns;
}
/**
* Returns the Set of learning {@link DistalDendrite}s
* @return
*/
public Set getLearningSegments() {
return learningSegments;
}
/**
* Sets the {@link Set} of learning segments
* @param segments
*/
public void setLearningSegments(Set segments) {
this.learningSegments = segments;
}
/**
* Returns the Set of active {@link DistalDendrite}s
* @return
*/
public Set getActiveSegments() {
return activeSegments;
}
/**
* Sets the {@link Set} of active {@link Segment}s
* @param segments
*/
public void setActiveSegments(Set segments) {
this.activeSegments = segments;
}
/**
* Returns the mapping of Segments to active synapses in t-1
* @return
*/
public Map> getActiveSynapsesForSegment() {
return activeSynapsesForSegment;
}
/**
* Sets the mapping of {@link Segment}s to active {@link Synapse}s
* @param syns
*/
public void setActiveSynapsesForSegment(Map> syns) {
this.activeSynapsesForSegment = syns;
}
/**
* Returns the mapping of {@link Cell}s to their reverse mapped
* {@link Synapse}s.
*
* @param cell the {@link Cell} used as a key.
* @return the mapping of {@link Cell}s to their reverse mapped
* {@link Synapse}s.
*/
public Set getReceptorSynapses(Cell cell) {
if(cell == null) {
throw new IllegalArgumentException("Cell was null");
}
if(receptorSynapses == null) {
receptorSynapses = new LinkedHashMap>();
}
Set retVal = null;
if((retVal = receptorSynapses.get(cell)) == null) {
receptorSynapses.put(cell, retVal = new LinkedHashSet());
}
return retVal;
}
/**
* Returns the mapping of {@link Cell}s to their {@link DistalDendrite}s.
*
* @param cell the {@link Cell} used as a key.
* @return the mapping of {@link Cell}s to their {@link DistalDendrite}s.
*/
public List getSegments(Cell cell) {
if(cell == null) {
throw new IllegalArgumentException("Cell was null");
}
if(segments == null) {
segments = new LinkedHashMap>();
}
List retVal = null;
if((retVal = segments.get(cell)) == null) {
segments.put(cell, retVal = new ArrayList());
}
return retVal;
}
/**
* Returns the mapping of {@link DistalDendrite}s to their {@link Synapse}s.
*
* @param segment the {@link DistalDendrite} used as a key.
* @return the mapping of {@link DistalDendrite}s to their {@link Synapse}s.
*/
public List getSynapses(DistalDendrite segment) {
if(segment == null) {
throw new IllegalArgumentException("Segment was null");
}
if(synapses == null) {
synapses = new LinkedHashMap>();
}
List retVal = null;
if((retVal = synapses.get(segment)) == null) {
synapses.put(segment, retVal = new ArrayList());
}
return retVal;
}
/**
* Returns the mapping of {@link ProximalDendrite}s to their {@link Synapse}s.
*
* @param segment the {@link ProximalDendrite} used as a key.
* @return the mapping of {@link ProximalDendrite}s to their {@link Synapse}s.
*/
public List getSynapses(ProximalDendrite segment) {
if(segment == null) {
throw new IllegalArgumentException("Segment was null");
}
if(synapses == null) {
synapses = new LinkedHashMap>();
}
List retVal = null;
if((retVal = synapses.get(segment)) == null) {
synapses.put(segment, retVal = new ArrayList());
}
return retVal;
}
/**
* Returns the column at the specified index.
* @param index
* @return
*/
public Column getColumn(int index) {
return memory.getObject(index);
}
/**
* Sets the number of {@link Column}.
*
* @param columnDimensions
*/
public void setColumnDimensions(int[] columnDimensions) {
this.columnDimensions = columnDimensions;
}
/**
* Gets the number of {@link Column}.
*
* @return columnDimensions
*/
public int[] getColumnDimensions() {
return this.columnDimensions;
}
/**
* A list representing the dimensions of the input
* vector. Format is [height, width, depth, ...], where
* each value represents the size of the dimension. For a
* topology of one dimension with 100 inputs use 100, or
* [100]. For a two dimensional topology of 10x5 use
* [10,5].
*
* @param inputDimensions
*/
public void setInputDimensions(int[] inputDimensions) {
this.inputDimensions = inputDimensions;
}
/**
* Returns the configured input dimensions
*
* @return the configured input dimensions
* @see {@link #setInputDimensions(int[])}
*/
public int[] getInputDimensions() {
return inputDimensions;
}
/**
* Sets the number of {@link Cell}s per {@link Column}
* @param cellsPerColumn
*/
public void setCellsPerColumn(int cellsPerColumn) {
this.cellsPerColumn = cellsPerColumn;
}
/**
* Gets the number of {@link Cells} per {@link Column}.
*
* @return cellsPerColumn
*/
public int getCellsPerColumn() {
return this.cellsPerColumn;
}
/**
* Sets the activation threshold.
*
* If the number of active connected synapses on a segment
* is at least this threshold, the segment is said to be active.
*
* @param activationThreshold
*/
public void setActivationThreshold(int activationThreshold) {
this.activationThreshold = activationThreshold;
}
/**
* Returns the activation threshold.
* @return
*/
public int getActivationThreshold() {
return activationThreshold;
}
/**
* Radius around cell from which it can
* sample to form distal dendrite connections.
*
* @param learningRadius
*/
public void setLearningRadius(int learningRadius) {
this.learningRadius = learningRadius;
}
/**
* Returns the learning radius.
* @return
*/
public int getLearningRadius() {
return learningRadius;
}
/**
* If the number of synapses active on a segment is at least this
* threshold, it is selected as the best matching
* cell in a bursting column.
*
* @param minThreshold
*/
public void setMinThreshold(int minThreshold) {
this.minThreshold = minThreshold;
}
/**
* Returns the minimum threshold of active synapses to be picked as best.
* @return
*/
public int getMinThreshold() {
return minThreshold;
}
/**
* The maximum number of synapses added to a segment during learning.
*
* @param maxNewSynapseCount
*/
public void setMaxNewSynapseCount(int maxNewSynapseCount) {
this.maxNewSynapseCount = maxNewSynapseCount;
}
/**
* Returns the maximum number of synapses added to a segment during
* learning.
*
* @return
*/
public int getMaxNewSynapseCount() {
return maxNewSynapseCount;
}
/**
* Initial permanence of a new synapse
*
* @param
*/
public void setInitialPermanence(double initialPermanence) {
this.initialPermanence = initialPermanence;
}
/**
* Returns the initial permanence setting.
* @return
*/
public double getInitialPermanence() {
return initialPermanence;
}
/**
* If the permanence value for a synapse
* is greater than this value, it is said
* to be connected.
*
* @param connectedPermanence
*/
public void setConnectedPermanence(double connectedPermanence) {
this.connectedPermanence = connectedPermanence;
}
/**
* If the permanence value for a synapse
* is greater than this value, it is said
* to be connected.
*
* @return
*/
public double getConnectedPermanence() {
return connectedPermanence;
}
/**
* Amount by which permanences of synapses
* are incremented during learning.
*
* @param permanenceIncrement
*/
public void setPermanenceIncrement(double permanenceIncrement) {
this.permanenceIncrement = permanenceIncrement;
}
/**
* Amount by which permanences of synapses
* are incremented during learning.
*
* @param permanenceIncrement
*/
public double getPermanenceIncrement() {
return this.permanenceIncrement;
}
/**
* Amount by which permanences of synapses
* are decremented during learning.
*
* @param permanenceDecrement
*/
public void setPermanenceDecrement(double permanenceDecrement) {
this.permanenceDecrement = permanenceDecrement;
}
/**
* Amount by which permanences of synapses
* are decremented during learning.
*
* @param permanenceDecrement
*/
public double getPermanenceDecrement() {
return this.permanenceDecrement;
}
/**
* Converts a {@link Collection} of {@link Cell}s to a list
* of cell indexes.
*
* @param cells
* @return
*/
public static List asCellIndexes(Collection cells) {
List ints = new ArrayList();
for(Cell cell : cells) {
ints.add(cell.getIndex());
}
return ints;
}
/**
* Converts a {@link Collection} of {@link Columns}s to a list
* of column indexes.
*
* @param columns
* @return
*/
public static List asColumnIndexes(Collection columns) {
List ints = new ArrayList();
for(Column col : columns) {
ints.add(col.getIndex());
}
return ints;
}
/**
* Returns a list of the {@link Cell}s specified.
* @param cells the indexes of the {@link Cell}s to return
* @return the specified list of cells
*/
public List asCellObjects(Collection cells) {
List objs = new ArrayList();
for(int i : cells) {
objs.add(this.cells[i]);
}
return objs;
}
/**
* Returns a list of the {@link Column}s specified.
* @param cols the indexes of the {@link Column}s to return
* @return the specified list of columns
*/
public List asColumnObjects(Collection cols) {
List objs = new ArrayList();
for(int i : cols) {
objs.add(this.memory.getObject(i));
}
return objs;
}
/**
* Returns a {@link Set} view of the {@link Column}s specified by
* the indexes passed in.
*
* @param indexes the indexes of the Columns to return
* @return a set view of the specified columns
*/
public LinkedHashSet getColumnSet(int[] indexes) {
LinkedHashSet retVal = new LinkedHashSet();
for(int i = 0;i < indexes.length;i++) {
retVal.add(memory.getObject(indexes[i]));
}
return retVal;
}
/**
* Returns a {@link List} view of the {@link Column}s specified by
* the indexes passed in.
*
* @param indexes the indexes of the Columns to return
* @return a List view of the specified columns
*/
public List getColumnList(int[] indexes) {
List retVal = new ArrayList();
for(int i = 0;i < indexes.length;i++) {
retVal.add(memory.getObject(indexes[i]));
}
return retVal;
}
}
| | | | | | | | | | | | | | | | | | | | | | |
© 2015 - 2025 Weber Informatics LLC | Privacy Policy