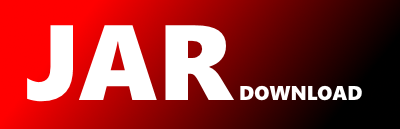
org.numenta.nupic.research.TemporalMemory Maven / Gradle / Ivy
Go to download
Show more of this group Show more artifacts with this name
Show all versions of htm.java Show documentation
Show all versions of htm.java Show documentation
The Java version of Numenta's HTM technology
/* ---------------------------------------------------------------------
* Numenta Platform for Intelligent Computing (NuPIC)
* Copyright (C) 2014, Numenta, Inc. Unless you have an agreement
* with Numenta, Inc., for a separate license for this software code, the
* following terms and conditions apply:
*
* This program is free software: you can redistribute it and/or modify
* it under the terms of the GNU General Public License version 3 as
* published by the Free Software Foundation.
*
* This program is distributed in the hope that it will be useful,
* but WITHOUT ANY WARRANTY; without even the implied warranty of
* MERCHANTABILITY or FITNESS FOR A PARTICULAR PURPOSE.
* See the GNU General Public License for more details.
*
* You should have received a copy of the GNU General Public License
* along with this program. If not, see http://www.gnu.org/licenses.
*
* http://numenta.org/licenses/
* ---------------------------------------------------------------------
*/
package org.numenta.nupic.research;
import java.util.ArrayList;
import java.util.LinkedHashMap;
import java.util.LinkedHashSet;
import java.util.List;
import java.util.Map;
import java.util.Set;
import org.numenta.nupic.Connections;
import org.numenta.nupic.model.Cell;
import org.numenta.nupic.model.Column;
import org.numenta.nupic.model.DistalDendrite;
import org.numenta.nupic.model.Synapse;
import org.numenta.nupic.util.SparseObjectMatrix;
/**
* Temporal Memory implementation in Java
*
* @author Chetan Surpur
* @author David Ray
*/
public class TemporalMemory {
/**
* Constructs a new {@code TemporalMemory}
*/
public TemporalMemory() {}
/**
* Uses the specified {@link Connections} object to Build the structural
* anatomy needed by this {@code TemporalMemory} to implement its algorithms.
*
* The connections object holds the {@link Column} and {@link Cell} infrastructure,
* and is used by both the {@link SpatialPooler} and {@link TemporalMemory}. Either of
* these can be used separately, and therefore this Connections object may have its
* Columns and Cells initialized by either the init method of the SpatialPooler or the
* init method of the TemporalMemory. We check for this so that complete initialization
* of both Columns and Cells occurs, without either being redundant (initialized more than
* once). However, {@link Cell}s only get created when initializing a TemporalMemory, because
* they are not used by the SpatialPooler.
*
* @param c {@link Connections} object
*/
public void init(Connections c) {
SparseObjectMatrix matrix = c.getMemory() == null ?
new SparseObjectMatrix(c.getColumnDimensions()) :
c.getMemory();
c.setMemory(matrix);
int numColumns = matrix.getMaxIndex() + 1;
int cellsPerColumn = c.getCellsPerColumn();
Cell[] cells = new Cell[numColumns * cellsPerColumn];
//Used as flag to determine if Column objects have been created.
Column colZero = matrix.getObject(0);
for(int i = 0;i < numColumns;i++) {
Column column = colZero == null ?
new Column(cellsPerColumn, i) : matrix.getObject(i);
for(int j = 0;j < cellsPerColumn;j++) {
cells[i * cellsPerColumn + j] = column.getCell(j);
}
//If columns have not been previously configured
if(colZero == null) matrix.set(i, column);
}
//Only the TemporalMemory initializes cells so no need to test
c.setCells(cells);
}
/////////////////////////// CORE FUNCTIONS /////////////////////////////
/**
* Feeds input record through TM, performing inferencing and learning
*
* @param connections the connection memory
* @param activeColumns direct proximal dendrite input
* @param learn learning mode flag
* @return {@link ComputeCycle} container for one cycle of inference values.
*/
public ComputeCycle compute(Connections connections, int[] activeColumns, boolean learn) {
ComputeCycle result = computeFn(connections, connections.getColumnSet(activeColumns), new LinkedHashSet(connections.getPredictiveCells()),
new LinkedHashSet(connections.getActiveSegments()), new LinkedHashMap>(connections.getActiveSynapsesForSegment()),
new LinkedHashSet(connections.getWinnerCells()), learn);
connections.setActiveCells(result.activeCells());
connections.setWinnerCells(result.winnerCells());
connections.setPredictiveCells(result.predictiveCells());
connections.setSuccessfullyPredictedColumns(result.successfullyPredictedColumns());
connections.setActiveSegments(result.activeSegments());
connections.setLearningSegments(result.learningSegments());
connections.setActiveSynapsesForSegment(result.activeSynapsesForSegment());
return result;
}
/**
* Functional version of {@link #compute(int[], boolean)}.
* This method is stateless and concurrency safe.
*
* @param c {@link Connections} object containing state of memory members
* @param activeColumns proximal dendrite input
* @param prevPredictiveCells cells predicting in t-1
* @param prevActiveSegments active segments in t-1
* @param prevActiveSynapsesForSegment {@link Synapse}s active in t-1
* @param prevWinnerCells ` previous winners
* @param learn whether mode is "learning" mode
* @return
*/
public ComputeCycle computeFn(Connections c, Set activeColumns, Set prevPredictiveCells, Set prevActiveSegments,
Map> prevActiveSynapsesForSegment, Set prevWinnerCells, boolean learn) {
ComputeCycle cycle = new ComputeCycle();
activateCorrectlyPredictiveCells(cycle, prevPredictiveCells, activeColumns);
burstColumns(cycle, c, activeColumns, cycle.successfullyPredictedColumns, prevActiveSynapsesForSegment);
if(learn) {
learnOnSegments(c, prevActiveSegments, cycle.learningSegments, prevActiveSynapsesForSegment, cycle.winnerCells, prevWinnerCells);
}
cycle.activeSynapsesForSegment = computeActiveSynapses(c, cycle.activeCells);
computePredictiveCells(c, cycle, cycle.activeSynapsesForSegment);
return cycle;
}
/**
* Phase 1: Activate the correctly predictive cells
*
* Pseudocode:
*
* - for each previous predictive cell
* - if in active column
* - mark it as active
* - mark it as winner cell
* - mark column as predicted
*
* @param c ComputeCycle interim values container
* @param prevPredictiveCells predictive {@link Cell}s predictive cells in t-1
* @param activeColumns active columns in t
*/
public void activateCorrectlyPredictiveCells(ComputeCycle c, Set prevPredictiveCells, Set activeColumns) {
for(Cell cell : prevPredictiveCells) {
Column column = cell.getParentColumn();
if(activeColumns.contains(column)) {
c.activeCells.add(cell);
c.winnerCells.add(cell);
c.successfullyPredictedColumns.add(column);
}
}
}
/**
* Phase 2: Burst unpredicted columns.
*
* Pseudocode:
*
* - for each unpredicted active column
* - mark all cells as active
* - mark the best matching cell as winner cell
* - (learning)
* - if it has no matching segment
* - (optimization) if there are previous winner cells
* - add a segment to it
* - mark the segment as learning
*
* @param cycle ComputeCycle interim values container
* @param c Connections temporal memory state
* @param activeColumns active columns in t
* @param predictedColumns predicted columns in t
* @param prevActiveSynapsesForSegment LinkedHashMap of previously active segments which
* have had synapses marked as active in t-1
*/
public void burstColumns(ComputeCycle cycle, Connections c, Set activeColumns, Set predictedColumns,
Map> prevActiveSynapsesForSegment) {
activeColumns.removeAll(predictedColumns);
for(Column column : activeColumns) {
List cells = column.getCells();
cycle.activeCells.addAll(cells);
Object[] bestSegmentAndCell = getBestMatchingCell(c, column, prevActiveSynapsesForSegment);
DistalDendrite bestSegment = (DistalDendrite)bestSegmentAndCell[0];
Cell bestCell = (Cell)bestSegmentAndCell[1];
if(bestCell != null) {
cycle.winnerCells.add(bestCell);
}
int segmentCounter = c.getSegmentCount();
if(bestSegment == null) {
bestSegment = bestCell.createSegment(c, segmentCounter);
c.setSegmentCount(segmentCounter + 1);
}
cycle.learningSegments.add(bestSegment);
}
}
/**
* Phase 3: Perform learning by adapting segments.
*
* Pseudocode:
*
* - (learning) for each previously active or learning segment
* - if learning segment or from winner cell
* - strengthen active synapses
* - weaken inactive synapses
* - if learning segment
* - add some synapses to the segment
* - sub sample from previous winner cells
*
*
* @param c the Connections state of the temporal memory
* @param prevActiveSegments the Set of segments active in the previous cycle.
* @param learningSegments the Set of segments marked as learning {@link #burstColumns(ComputeCycle, Connections, Set, Set, Map)}
* @param prevActiveSynapseSegments the map of segments which were previously active to their associated {@link Synapse}s.
* @param winnerCells the Set of all winning cells ({@link Cell}s with the most active synapses)
* @param prevWinnerCells the Set of cells which were winners during the last compute cycle
*/
public void learnOnSegments(Connections c, Set prevActiveSegments, Set learningSegments,
Map> prevActiveSynapseSegments, Set winnerCells, Set prevWinnerCells) {
double permanenceIncrement = c.getPermanenceIncrement();
double permanenceDecrement = c.getPermanenceDecrement();
List prevAndLearning = new ArrayList(prevActiveSegments);
prevAndLearning.addAll(learningSegments);
for(DistalDendrite dd : prevAndLearning) {
boolean isLearningSegment = learningSegments.contains(dd);
boolean isFromWinnerCell = winnerCells.contains(dd.getParentCell());
Set activeSynapses = dd.getConnectedActiveSynapses(prevActiveSynapseSegments, 0);
if(isLearningSegment || isFromWinnerCell) {
dd.adaptSegment(c, activeSynapses, permanenceIncrement, permanenceDecrement);
}
int synapseCounter = c.getSynapseCount();
int n = c.getMaxNewSynapseCount() - activeSynapses.size();
if(isLearningSegment && n > 0) {
Set learnCells = dd.pickCellsToLearnOn(c, n, prevWinnerCells, c.getRandom());
for(Cell sourceCell : learnCells) {
dd.createSynapse(c, sourceCell, c.getInitialPermanence(), synapseCounter);
synapseCounter += 1;
}
c.setSynapseCount(synapseCounter);
}
}
}
/**
* Phase 4: Compute predictive cells due to lateral input on distal dendrites.
*
* Pseudocode:
*
* - for each distal dendrite segment with activity >= activationThreshold
* - mark the segment as active
* - mark the cell as predictive
*
* @param c the Connections state of the temporal memory
* @param cycle the state during the current compute cycle
* @param activeSegments
*/
public void computePredictiveCells(Connections c, ComputeCycle cycle, Map> activeDendrites) {
for(DistalDendrite dd : activeDendrites.keySet()) {
Set connectedActive = dd.getConnectedActiveSynapses(activeDendrites, c.getConnectedPermanence());
if(connectedActive.size() >= c.getActivationThreshold()) {
cycle.activeSegments.add(dd);
cycle.predictiveCells.add(dd.getParentCell());
}
}
}
/**
* Forward propagates activity from active cells to the synapses that touch
* them, to determine which synapses are active.
*
* @param c the connections state of the temporal memory
* @param cellsActive
* @return
*/
public Map> computeActiveSynapses(Connections c, Set cellsActive) {
Map> activesSynapses = new LinkedHashMap>();
for(Cell cell : cellsActive) {
for(Synapse s : cell.getReceptorSynapses(c)) {
Set set = null;
if((set = activesSynapses.get(s.getSegment())) == null) {
activesSynapses.put((DistalDendrite)s.getSegment(), set = new LinkedHashSet());
}
set.add(s);
}
}
return activesSynapses;
}
/**
* Called to start the input of a new sequence.
*
* @param connections the Connections state of the temporal memory
*/
public void reset(Connections connections) {
connections.getActiveCells().clear();
connections.getPredictiveCells().clear();
connections.getActiveSegments().clear();
connections.getActiveSynapsesForSegment().clear();
connections.getWinnerCells().clear();
}
/////////////////////////// HELPER FUNCTIONS ///////////////////////////
/**
* Gets the cell with the best matching segment
* (see `TM.getBestMatchingSegment`) that has the largest number of active
* synapses of all best matching segments.
*
* @param c encapsulated memory and state
* @param column {@link Column} within which to search for best cell
* @param prevActiveSynapsesForSegment a {@link DistalDendrite}'s previously active {@link Synapse}s
* @return an object array whose first index contains a segment, and the second contains a cell
*/
public Object[] getBestMatchingCell(Connections c, Column column, Map> prevActiveSynapsesForSegment) {
Object[] retVal = new Object[2];
Cell bestCell = null;
DistalDendrite bestSegment = null;
int maxSynapses = 0;
for(Cell cell : column.getCells()) {
DistalDendrite dd = getBestMatchingSegment(c, cell, prevActiveSynapsesForSegment);
if(dd != null) {
Set connectedActiveSynapses = dd.getConnectedActiveSynapses(prevActiveSynapsesForSegment, 0);
if(connectedActiveSynapses.size() > maxSynapses) {
maxSynapses = connectedActiveSynapses.size();
bestCell = cell;
bestSegment = dd;
}
}
}
if(bestCell == null) {
bestCell = column.getLeastUsedCell(c, c.getRandom());
}
retVal[0] = bestSegment;
retVal[1] = bestCell;
return retVal;
}
/**
* Gets the segment on a cell with the largest number of activate synapses,
* including all synapses with non-zero permanences.
*
* @param c encapsulated memory and state
* @param column {@link Column} within which to search for best cell
* @param activeSynapseSegments a {@link DistalDendrite}'s active {@link Synapse}s
* @return the best segment
*/
public DistalDendrite getBestMatchingSegment(Connections c, Cell cell, Map> activeSynapseSegments) {
int maxSynapses = c.getMinThreshold();
DistalDendrite bestSegment = null;
for(DistalDendrite dd : cell.getSegments(c)) {
Set activeSyns = dd.getConnectedActiveSynapses(activeSynapseSegments, 0);
if(activeSyns.size() >= maxSynapses) {
maxSynapses = activeSyns.size();
bestSegment = dd;
}
}
return bestSegment;
}
/**
* Returns the column index given the cells per column and
* the cell index passed in.
*
* @param c {@link Connections} memory
* @param cellIndex the index where the requested cell resides
* @return
*/
protected int columnForCell(Connections c, int cellIndex) {
return cellIndex / c.getCellsPerColumn();
}
/**
* Returns the cell at the specified index.
* @param index
* @return
*/
public Cell getCell(Connections c, int index) {
return c.getCells()[index];
}
/**
* Returns a {@link LinkedHashSet} of {@link Cell}s from a
* sorted array of cell indexes.
*
* @param`c the {@link Connections} object
* @param cellIndexes indexes of the {@link Cell}s to return
* @return
*/
public LinkedHashSet getCells(Connections c, int[] cellIndexes) {
LinkedHashSet cellSet = new LinkedHashSet();
for(int cell : cellIndexes) {
cellSet.add(getCell(c, cell));
}
return cellSet;
}
/**
* Returns a {@link LinkedHashSet} of {@link Column}s from a
* sorted array of Column indexes.
*
* @param cellIndexes indexes of the {@link Column}s to return
* @return
*/
public LinkedHashSet getColumns(Connections c, int[] columnIndexes) {
return c.getColumnSet(columnIndexes);
}
}
| | | | | | | | | | | | |
© 2015 - 2025 Weber Informatics LLC | Privacy Policy