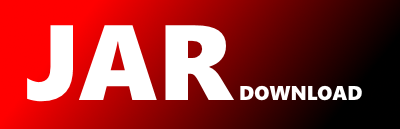
org.numenta.nupic.algorithms.Anomaly Maven / Gradle / Ivy
Go to download
Show more of this group Show more artifacts with this name
Show all versions of htm.java Show documentation
Show all versions of htm.java Show documentation
The Java version of Numenta's HTM technology
The newest version!
/* ---------------------------------------------------------------------
* Numenta Platform for Intelligent Computing (NuPIC)
* Copyright (C) 2014, Numenta, Inc. Unless you have an agreement
* with Numenta, Inc., for a separate license for this software code, the
* following terms and conditions apply:
*
* This program is free software: you can redistribute it and/or modify
* it under the terms of the GNU Affero Public License version 3 as
* published by the Free Software Foundation.
*
* This program is distributed in the hope that it will be useful,
* but WITHOUT ANY WARRANTY; without even the implied warranty of
* MERCHANTABILITY or FITNESS FOR A PARTICULAR PURPOSE.
* See the GNU Affero Public License for more details.
*
* You should have received a copy of the GNU Affero Public License
* along with this program. If not, see http://www.gnu.org/licenses.
*
* http://numenta.org/licenses/
* ---------------------------------------------------------------------
*/
package org.numenta.nupic.algorithms;
import java.util.HashMap;
import java.util.List;
import java.util.Map;
import org.numenta.nupic.model.Persistable;
import org.numenta.nupic.util.ArrayUtils;
import gnu.trove.list.TDoubleList;
import gnu.trove.list.array.TDoubleArrayList;
/**
* This module analyzes and estimates the distribution of averaged anomaly scores
* from a CLA model. Given a new anomaly score `s`, estimates `P(score >= s)`.
*
* The number `P(score >= s)` represents the likelihood of the current state of
* predictability. For example, a likelihood of 0.01 or 1% means we see this much
* predictability about one out of every 100 records. The number is not as unusual
* as it seems. For records that arrive every minute, this means once every hour
* and 40 minutes. A likelihood of 0.0001 or 0.01% means we see it once out of
* 10,000 records, or about once every 7 days.
*
* USAGE
* -----
*
* The {@code Anomaly} base class follows the factory pattern and can construct an
* appropriately configured anomaly calculator by invoking the following:
*
*
* Map params = new HashMap<>();
* params.put(KEY_MODE, Mode.LIKELIHOOD); // May be Mode.PURE or Mode.WEIGHTED
* params.put(KEY_USE_MOVING_AVG, true); // Instructs the Anomaly class to compute moving average
* params.put(KEY_WINDOW_SIZE, 10); // #of inputs over which to compute the moving average
* params.put(KEY_IS_WEIGHTED, true); // Use a weighted moving average or not
*
* // Instantiate the Anomaly computer
* Anomaly anomalyComputer = Anomaly.create(params); // Returns the appropriate Anomaly
* // implementation.
* int[] actual = array of input columns at time t
* int[] predicted = array of predicted columns for t+1
* double anomaly = an.compute(
* actual,
* predicted,
* 0 (inputValue = OPTIONAL, needed for likelihood calcs),
* timestamp);
*
* double anomalyProbability = anomalyComputer.anomalyProbability(
* inputValue, anomaly, timestamp);
*
*
* Raw functions
* -------------
*
* There are two lower level functions, estimateAnomalyLikelihoods and
* updateAnomalyLikelihoods. The details of these are described by the method docs.
*
* For more information please see: {@link AnomalyTest} and {@link AnomalyLikelihoodTest}
*
* @author Numenta
* @author David Ray
* @see AnomalyTest
* @see AnomalyLikelihoodTest
*/
public abstract class Anomaly implements Persistable {
private static final long serialVersionUID = 1L;
/** Modes to use for factory creation method */
public enum Mode { PURE, LIKELIHOOD, WEIGHTED };
// Instantiation keys
public static final int VALUE_NONE = -1;
public static final String KEY_MODE = "mode".intern();
public static final String KEY_LEARNING_PERIOD = "claLearningPeriod";
public static final String KEY_ESTIMATION_SAMPLES = "estimationSamples";
public static final String KEY_USE_MOVING_AVG = "useMovingAverage";
public static final String KEY_WINDOW_SIZE = "windowSize".intern();
public static final String KEY_IS_WEIGHTED = "isWeighted";
// Configs
public static final String KEY_DIST = "distribution".intern();
public static final String KEY_MVG_AVG = "movingAverage".intern();
public static final String KEY_HIST_LIKE = "historicalLikelihoods".intern();
public static final String KEY_HIST_VALUES = "historicalValues".intern();
public static final String KEY_TOTAL = "total".intern();
// Computational argument keys
public final static String KEY_MEAN = "mean".intern();
public final static String KEY_STDEV = "stdev".intern();
public final static String KEY_VARIANCE = "variance".intern();
protected MovingAverage movingAverage;
protected boolean useMovingAverage;
/**
* Constructs a new {@code Anomaly}
*/
public Anomaly() {
this(false, -1);
}
/**
* Constructs a new {@code Anomaly}
*
* @param useMovingAverage indicates whether to apply and store a moving average
* @param windowSize size of window to average over
*/
protected Anomaly(boolean useMovingAverage, int windowSize) {
this.useMovingAverage = useMovingAverage;
if(this.useMovingAverage) {
if(windowSize < 1) {
throw new IllegalArgumentException(
"Window size must be > 0, when using moving average.");
}
movingAverage = new MovingAverage(null, windowSize);
}
}
/**
* Convenience method to create a simplistic Anomaly computer in
* {@link Mode#PURE}
*
* @return
*/
public static Anomaly create() {
Map params = new HashMap<>();
params.put(KEY_MODE, Mode.PURE);
return create(params);
}
/**
* Returns an {@code Anomaly} configured to execute the type
* of calculation specified by the {@link Mode}, and whether or
* not to apply a moving average.
*
* Must have one of "MODE" = {@link Mode#LIKELIHOOD}, {@link Mode#PURE}, {@link Mode#WEIGHTED}
*
* @param p Map
* @return
*/
public static Anomaly create(Map params) {
boolean useMovingAvg = (boolean)params.getOrDefault(KEY_USE_MOVING_AVG, false);
int windowSize = (int)params.getOrDefault(KEY_WINDOW_SIZE, -1);
if(useMovingAvg && windowSize < 1) {
throw new IllegalArgumentException("windowSize must be > 0, when using moving average.");
}
Mode mode = (Mode)params.get(KEY_MODE);
if(mode == null) {
throw new IllegalArgumentException("MODE cannot be null.");
}
switch(mode) {
case PURE: return new Anomaly(useMovingAvg, windowSize) {
private static final long serialVersionUID = 1L;
@Override
public double compute(int[] activeColumns, int[] predictedColumns, double inputValue, long timestamp) {
double retVal = computeRawAnomalyScore(activeColumns, predictedColumns);
if(this.useMovingAverage) {
retVal = movingAverage.next(retVal);
}
return retVal;
}
};
case LIKELIHOOD:
case WEIGHTED: {
boolean isWeighted = (boolean)params.getOrDefault(KEY_IS_WEIGHTED, false);
int claLearningPeriod = (int)params.getOrDefault(KEY_LEARNING_PERIOD, VALUE_NONE);
int estimationSamples = (int)params.getOrDefault(KEY_ESTIMATION_SAMPLES, VALUE_NONE);
return new AnomalyLikelihood(useMovingAvg, windowSize, isWeighted, claLearningPeriod, estimationSamples);
}
default: return null;
}
}
/**
* The raw anomaly score is the fraction of active columns not predicted.
*
* @param activeColumns an array of active column indices
* @param prevPredictedColumns array of column indices predicted in the
* previous step
* @return anomaly score 0..1
*/
public static double computeRawAnomalyScore(int[] activeColumns, int[] prevPredictedColumns) {
double score = 0;
int nActiveColumns = activeColumns.length;
if(nActiveColumns > 0) {
// Test whether each element of a 1-D array is also present in a second
// array. Sum to get the total # of columns that are active and were
// predicted.
score = ArrayUtils.in1d(activeColumns, prevPredictedColumns).length;
// Get the percent of active columns that were NOT predicted, that is
// our anomaly score.
score = (nActiveColumns - score) / (double)nActiveColumns;
} else {
score = 0.0d;
}
return score;
}
/**
* Compute the anomaly score as the percent of active columns not predicted.
*
* @param activeColumns array of active column indices
* @param predictedColumns array of columns indices predicted in this step
* (used for anomaly in step T+1)
* @param inputValue (optional) value of current input to encoders
* (eg "cat" for category encoder)
* (used in anomaly-likelihood)
* @param timestamp timestamp: (optional) date timestamp when the sample occurred
* (used in anomaly-likelihood)
* @return
*/
public abstract double compute(int[] activeColumns, int[] predictedColumns, double inputValue, long timestamp);
//////////////////////////////////////////////////////////////////////////////////////
// Inner Class Definitions //
//////////////////////////////////////////////////////////////////////////////////////
/**
* Container to hold interim {@link AnomalyLikelihood} calculations.
*
* @author David Ray
* @see AnomalyLikelihood
* @see MovingAverage
*/
public class AveragedAnomalyRecordList implements Persistable {
private static final long serialVersionUID = 1L;
public List averagedRecords;
public TDoubleList historicalValues;
public double total;
/**
* Constructs a new {@code AveragedAnomalyRecordList}
*
* @param averagedRecords List of samples which are { timestamp, average, value } at a data point
* @param historicalValues List of values of a given window size (moving average grouping)
* @param total Sum of all values in the series
*/
public AveragedAnomalyRecordList(List averagedRecords, TDoubleList historicalValues, double total) {
this.averagedRecords = averagedRecords;
this.historicalValues = historicalValues;
this.total = total;
}
/**
* Returns a list of the averages in the contained averaged record list.
* @return
*/
public TDoubleList getMetrics() {
TDoubleList retVal = new TDoubleArrayList();
for(Sample s : averagedRecords) {
retVal.add(s.score);
}
return retVal;
}
/**
* Returns a list of the sample values in the contained averaged record list.
* @return
*/
public TDoubleList getSamples() {
TDoubleList retVal = new TDoubleArrayList();
for(Sample s : averagedRecords) {
retVal.add(s.value);
}
return retVal;
}
/**
* Returns the size of the count of averaged records (i.e. {@link Sample}s)
* @return
*/
public int size() {
return averagedRecords.size(); //let fail if null
}
@Override
public int hashCode() {
final int prime = 31;
int result = 1;
result = prime * result + ((averagedRecords == null) ? 0 : averagedRecords.hashCode());
result = prime * result + ((historicalValues == null) ? 0 : historicalValues.hashCode());
long temp;
temp = Double.doubleToLongBits(total);
result = prime * result + (int)(temp ^ (temp >>> 32));
return result;
}
@Override
public boolean equals(Object obj) {
if(this == obj)
return true;
if(obj == null)
return false;
if(getClass() != obj.getClass())
return false;
AveragedAnomalyRecordList other = (AveragedAnomalyRecordList)obj;
if(averagedRecords == null) {
if(other.averagedRecords != null)
return false;
} else if(!averagedRecords.equals(other.averagedRecords))
return false;
if(historicalValues == null) {
if(other.historicalValues != null)
return false;
} else if(!historicalValues.equals(other.historicalValues))
return false;
if(Double.doubleToLongBits(total) != Double.doubleToLongBits(other.total))
return false;
return true;
}
}
}
© 2015 - 2025 Weber Informatics LLC | Privacy Policy