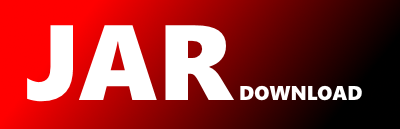
org.numenta.nupic.algorithms.AnomalyLikelihood Maven / Gradle / Ivy
Go to download
Show more of this group Show more artifacts with this name
Show all versions of htm.java Show documentation
Show all versions of htm.java Show documentation
The Java version of Numenta's HTM technology
The newest version!
/* ---------------------------------------------------------------------
* Numenta Platform for Intelligent Computing (NuPIC)
* Copyright (C) 2014, Numenta, Inc. Unless you have an agreement
* with Numenta, Inc., for a separate license for this software code, the
* following terms and conditions apply:
*
* This program is free software: you can redistribute it and/or modify
* it under the terms of the GNU Affero Public License version 3 as
* published by the Free Software Foundation.
*
* This program is distributed in the hope that it will be useful,
* but WITHOUT ANY WARRANTY; without even the implied warranty of
* MERCHANTABILITY or FITNESS FOR A PARTICULAR PURPOSE.
* See the GNU Affero Public License for more details.
*
* You should have received a copy of the GNU Affero Public License
* along with this program. If not, see http://www.gnu.org/licenses.
*
* http://numenta.org/licenses/
* ---------------------------------------------------------------------
*/
package org.numenta.nupic.algorithms;
import java.util.ArrayList;
import java.util.Arrays;
import java.util.List;
import org.joda.time.DateTime;
import org.numenta.nupic.algorithms.MovingAverage.Calculation;
import org.numenta.nupic.util.ArrayUtils;
import org.numenta.nupic.util.NamedTuple;
import org.slf4j.Logger;
import org.slf4j.LoggerFactory;
import gnu.trove.list.TDoubleList;
import gnu.trove.list.array.TDoubleArrayList;
import gnu.trove.map.TObjectDoubleMap;
/**
* The anomaly likelihood computer.
*
* From {@link Anomaly}:
*
* This module analyzes and estimates the distribution of averaged anomaly scores
* from a CLA model. Given a new anomaly score `s`, estimates `P(score >= s)`.
*
* The number `P(score >= s)` represents the likelihood of the current state of
* predictability. For example, a likelihood of 0.01 or 1% means we see this much
* predictability about one out of every 100 records. The number is not as unusual
* as it seems. For records that arrive every minute, this means once every hour
* and 40 minutes. A likelihood of 0.0001 or 0.01% means we see it once out of
* 10,000 records, or about once every 7 days.
*
*
*
* USAGE FOR LOW-LEVEL FUNCTIONS
* -----------------------------
*
*
* There are two primary interface routines:
*
* estimateAnomalyLikelihoods: batch routine, called initially and once in a
* while
* updateAnomalyLikelihoods: online routine, called for every new data point
*
* 1. Initially::
*
* {@link AnomalyLikelihoodMetrics} { likelihoods, avgRecordList, estimatorParams } =
* {@link #estimateAnomalyLikelihoods(List, int, int)}
*
* 2. Whenever you get new data::
*
* {@link AnomalyLikelihoodMetrics} { likelihoods, avgRecordList, estimatorParams } =
* {@link #updateAnomalyLikelihoods(List, NamedTuple)}
*
* 3. And again (make sure you use the new estimatorParams (a.k.a {@link NamedTuple}) returned
* in the above {@link AnomalyLikelihoodMetrics} call to updateAnomalyLikelihoods!)
*
* {@link AnomalyLikelihoodMetrics} { likelihoods, avgRecordList, estimatorParams } =
* {@link #updateAnomalyLikelihoods(List, NamedTuple)}
*
* 4. Every once in a while update estimator with a lot of recent data
*
* {@link AnomalyLikelihoodMetrics} { likelihoods, avgRecordList, estimatorParams } =
* {@link #estimateAnomalyLikelihoods(List, int, int)}
*
*
*
* @author Numenta
* @author David Ray
* @see Anomaly
* @see AnomalyLikelihoodMetrics
* @see Sample
* @see AnomalyParams
* @see Statistic
* @see MovingAverage
*/
public class AnomalyLikelihood extends Anomaly {
private static final long serialVersionUID = 1L;
private static final Logger LOG = LoggerFactory.getLogger(AnomalyLikelihood.class);
private int claLearningPeriod = 300;
private int estimationSamples = 300;
private int probationaryPeriod;
private int iteration;
private int reestimationPeriod;
private boolean isWeighted;
private List historicalScores = new ArrayList<>();
private AnomalyParams distribution;
public AnomalyLikelihood(boolean useMovingAvg, int windowSize, boolean isWeighted, int claLearningPeriod, int estimationSamples) {
super(useMovingAvg, windowSize);
this.isWeighted = isWeighted;
this.claLearningPeriod = claLearningPeriod == VALUE_NONE ? this.claLearningPeriod : claLearningPeriod;
this.estimationSamples = estimationSamples == VALUE_NONE ? this.estimationSamples : estimationSamples;
this.probationaryPeriod = this.claLearningPeriod + this.estimationSamples;
// How often we re-estimate the Gaussian distribution. The ideal is to
// re-estimate every iteration but this is a performance hit. In general the
// system is not very sensitive to this number as long as it is small
// relative to the total number of records processed.
this.reestimationPeriod = 100;
}
/**
* Compute a log scale representation of the likelihood value. Since the
* likelihood computations return low probabilities that often go into four 9's
* or five 9's, a log value is more useful for visualization, thresholding,
* etc.
*
* @param likelihood
* @return
*/
public static double computeLogLikelihood(double likelihood) {
return Math.log(1.0000000001 - likelihood) / -23.02585084720009;
}
/**
* Return the probability that the current value plus anomaly score represents
* an anomaly given the historical distribution of anomaly scores. The closer
* the number is to 1, the higher the chance it is an anomaly.
*
* Given the current metric value, plus the current anomaly score, output the
* anomalyLikelihood for this record.
*
* @param value input value
* @param anomalyScore current anomaly score
* @param timestamp (optional) timestamp
* @return Given the current metric value, plus the current anomaly score, output the
* anomalyLikelihood for this record.
*/
public double anomalyProbability(double value, double anomalyScore, DateTime timestamp) {
if(timestamp == null) {
timestamp = new DateTime();
}
Sample dataPoint = new Sample(timestamp, value, anomalyScore);
double likelihoodRetval;
if(historicalScores.size() < probationaryPeriod) {
likelihoodRetval = 0.5;
}else{
if(distribution == null || iteration % reestimationPeriod == 0) {
this.distribution = estimateAnomalyLikelihoods(
historicalScores, 10, claLearningPeriod).getParams();
}
AnomalyLikelihoodMetrics metrics = updateAnomalyLikelihoods(Arrays.asList(dataPoint), this.distribution);
this.distribution = metrics.getParams();
likelihoodRetval = 1.0 - metrics.getLikelihoods()[0];
}
historicalScores.add(dataPoint);
this.iteration += 1;
return likelihoodRetval;
}
/**
* Given a series of anomaly scores, compute the likelihood for each score. This
* function should be called once on a bunch of historical anomaly scores for an
* initial estimate of the distribution. It should be called again every so often
* (say every 50 records) to update the estimate.
*
* @param anomalyScores
* @param averagingWindow
* @param skipRecords
* @return
*/
public AnomalyLikelihoodMetrics estimateAnomalyLikelihoods(List anomalyScores, int averagingWindow, int skipRecords) {
if(anomalyScores.size() == 0) {
throw new IllegalArgumentException("Must have at least one anomaly score.");
}
// Compute averaged anomaly scores
AveragedAnomalyRecordList records = anomalyScoreMovingAverage(anomalyScores, averagingWindow);
// Estimate the distribution of anomaly scores based on aggregated records
Statistic distribution;
if(records.averagedRecords.size() <= skipRecords) {
distribution = nullDistribution();
}else{
TDoubleList samples = records.getMetrics();
final int numRecordsToCopy = samples.size() - skipRecords;
distribution = estimateNormal(samples.toArray(skipRecords, numRecordsToCopy), true);
/* Taken from the Python Documentation
# HACK ALERT! The CLA model currently does not handle constant metric values
# very well (time of day encoder changes sometimes lead to unstable SDR's
# even though the metric is constant). Until this is resolved, we explicitly
# detect and handle completely flat metric values by reporting them as not
# anomalous.
*/
samples = records.getSamples();
Statistic metricDistribution = estimateNormal(samples.toArray(skipRecords, numRecordsToCopy), false);
if(metricDistribution.variance < 1.5e-5) {
distribution = nullDistribution();
}
}
// Estimate likelihoods based on this distribution
int i = 0;
double[] likelihoods = new double[records.averagedRecords.size()];
for(Sample sample : records.averagedRecords) {
likelihoods[i++] = normalProbability(sample.score, distribution);
}
// Filter likelihood values
double[] filteredLikelihoods = filterLikelihoods(likelihoods);
int len = likelihoods.length;
AnomalyParams params = new AnomalyParams(
new String[] { "distribution", "movingAverage", "historicalLikelihoods" },
distribution,
new MovingAverage(records.historicalValues, records.total, averagingWindow),
len > 0 ?
Arrays.copyOfRange(likelihoods, len - Math.min(averagingWindow, len), len) :
new double[0]);
if(LOG.isDebugEnabled()) {
LOG.debug(
"Discovered params={} Number of likelihoods:{} First 20 likelihoods:{}",
params, len, Arrays.copyOfRange(filteredLikelihoods, 0, 20));
}
return new AnomalyLikelihoodMetrics(filteredLikelihoods, records, params);
}
/**
* Compute updated probabilities for anomalyScores using the given params.
*
* @param anomalyScores a list of records. Each record is a list with a {@link Sample} containing the
following three elements: [timestamp, value, score]
* @param params Associative {@link NamedTuple} returned by the {@link AnomalyLikelihoodMetrics} from
* {@link #estimateAnomalyLikelihoods(List, int, int)}
* @return
*/
public AnomalyLikelihoodMetrics updateAnomalyLikelihoods(List anomalyScores, NamedTuple params) {
int anomalySize = anomalyScores.size();
if(LOG.isDebugEnabled()) {
LOG.debug("in updateAnomalyLikelihoods");
LOG.debug("Number of anomaly scores: {}", anomalySize);
LOG.debug("First 20: {}", anomalyScores.subList(0, Math.min(20, anomalySize)));
LOG.debug("Params: {}", params);
}
if(anomalyScores.size() == 0) {
throw new IllegalArgumentException("Must have at least one anomaly score.");
}
if(!isValidEstimatorParams(params)) {
throw new IllegalArgumentException("\"params\" is not a valid parameter structure");
}
double[] histLikelihoods;
if(!params.hasKey("historicalLikelihoods") ||
(histLikelihoods = (double[])params.get("historicalLikelihoods")) == null ||
histLikelihoods.length == 0) {
params = new NamedTuple(
new String[] { "distribution", "movingAverage", "historicalLikelihoods" },
params.get("distribution"),
params.get("movingAverage"),
histLikelihoods = new double[] { 1 });
}
// Compute moving averages of these new scores using the previous values
// as well as likelihood for these scores using the old estimator
MovingAverage mvgAvg = (MovingAverage)params.get("movingAverage");
TDoubleList historicalValues = mvgAvg.getSlidingWindow();
double total = mvgAvg.getTotal();
int windowSize = mvgAvg.getWindowSize();
List aggRecordList = new ArrayList<>(anomalySize);
double[] likelihoods = new double[anomalySize];
int i = 0;
for(Sample sample : anomalyScores) {
Calculation calc = MovingAverage.compute(historicalValues, total, sample.score, windowSize);
aggRecordList.add(
new Sample(
sample.date,
sample.value,
calc.getAverage()));
total = calc.getTotal();
likelihoods[i++] = normalProbability(calc.getAverage(), (Statistic)params.get("distribution"));
}
// Filter the likelihood values. First we prepend the historical likelihoods
// to the current set. Then we filter the values. We peel off the likelihoods
// to return and the last windowSize values to store for later.
double[] likelihoods2 = ArrayUtils.concat(histLikelihoods, likelihoods);
double[] filteredLikelihoods = filterLikelihoods(likelihoods2);
likelihoods = Arrays.copyOfRange(filteredLikelihoods, filteredLikelihoods.length - likelihoods.length, filteredLikelihoods.length);
double[] historicalLikelihoods = Arrays.copyOf(likelihoods2, likelihoods2.length - Math.min(windowSize, likelihoods2.length));
// Update the estimator
AnomalyParams newParams = new AnomalyParams(
new String[] { "distribution", "movingAverage", "historicalLikelihoods" },
params.get("distribution"),
new MovingAverage(historicalValues, total, windowSize),
historicalLikelihoods);
return new AnomalyLikelihoodMetrics(
likelihoods,
new AveragedAnomalyRecordList(aggRecordList, historicalValues, total),
newParams);
}
/**
* Filter the list of raw (pre-filtered) likelihoods so that we only preserve
* sharp increases in likelihood. 'likelihoods' can be a numpy array of floats or
* a list of floats.
*
* @param likelihoods
* @return
*/
public double[] filterLikelihoods(double[] likelihoods) {
return filterLikelihoods(likelihoods, 0.99999, 0.999);
}
/**
* Filter the list of raw (pre-filtered) likelihoods so that we only preserve
* sharp increases in likelihood. 'likelihoods' can be an array of floats or
* a list of floats.
*
* @param likelihoods
* @param redThreshold
* @param yellowThreshold
* @return
*/
public double[] filterLikelihoods(double[] likelihoods, double redThreshold, double yellowThreshold) {
redThreshold = 1.0 - redThreshold;
yellowThreshold = 1.0 - yellowThreshold;
// The first value is untouched
double[] filteredLikelihoods = new double[likelihoods.length];
filteredLikelihoods[0] = likelihoods[0];
for(int i = 0;i < likelihoods.length - 1;i++) {
double v = likelihoods[i + 1];
if(v <= redThreshold) {
// If value is in redzone
if(likelihoods[i] > redThreshold) {
// Previous value is not in redzone, so leave as-is
filteredLikelihoods[i + 1] = v;
}else{
filteredLikelihoods[i + 1] = yellowThreshold;
}
}else{
// Value is below the redzone, so leave as-is
filteredLikelihoods[i + 1] = v;
}
}
return filteredLikelihoods;
}
/**
* Given a list of anomaly scores return a list of averaged records.
* anomalyScores is assumed to be a list of records of the form:
*
* Sample:
* dt = Tuple(2013, 8, 10, 23, 0) --> Date Fields
* sample = (double) 6.0
* metric(avg) = (double) 1.0
*
*
* @param anomalyScores List of {@link Sample} objects (described contents above)
* @param windowSize Count of historical items over which to compute the average
*
* @return Each record in the returned list contains [datetime field, value, averaged score]
*/
public AveragedAnomalyRecordList anomalyScoreMovingAverage(List anomalyScores, int windowSize) {
TDoubleList historicalValues = new TDoubleArrayList();
double total = 0.0;
List averagedRecordList = new ArrayList();
for(Sample record : anomalyScores) {
////////////////////////////////////////////////////////////////////////////////////////////
// Python version has check for malformed records here, but can't happen in java version. //
////////////////////////////////////////////////////////////////////////////////////////////
Calculation calc = MovingAverage.compute(historicalValues, total, record.score, windowSize);
Sample avgRecord = new Sample(
record.date,
record.value,
calc.getAverage());
averagedRecordList.add(avgRecord);
total = calc.getTotal();
if(LOG.isDebugEnabled()) {
LOG.debug("Aggregating input record: {}, Result: {}", record, averagedRecordList.get(averagedRecordList.size() - 1));
}
}
return new AveragedAnomalyRecordList(averagedRecordList, historicalValues, total);
}
/**
* A Map containing the parameters of a normal distribution based on
* the sampleData.
*
* @param sampleData
* @param performLowerBoundCheck
* @return
*/
public Statistic estimateNormal(double[] sampleData, boolean performLowerBoundCheck) {
double d = ArrayUtils.average(sampleData);
double v = ArrayUtils.variance(sampleData, d);
if(performLowerBoundCheck) {
if(d < 0.03) {
d = 0.03;
}
if(v < 0.0003) {
v = 0.0003;
}
}
// Compute standard deviation
double s = v > 0 ? Math.sqrt(v) : 0.0;
return new Statistic(d, v, s);
}
/**
* Returns a distribution that is very broad and makes every anomaly
* score between 0 and 1 pretty likely
*
* @return
*/
public Statistic nullDistribution() {
if(LOG.isDebugEnabled()) {
LOG.debug("Returning nullDistribution");
}
return new Statistic(0.5, 1e6, 1e3);
}
/**
* Given the normal distribution specified in distributionParams, return
* the probability of getting samples > x
* This is essentially the Q-function
*
* @param x
* @param named
* @return
*/
public double normalProbability(double x, TObjectDoubleMap named) {
return normalProbability(x,
new Statistic(named.get(KEY_MEAN), named.get(KEY_VARIANCE), named.get(KEY_STDEV)));
}
/**
* Given the normal distribution specified in distributionParams, return
* the probability of getting samples > x
* This is essentially the Q-function
*
* @param x
* @param named
* @return
*/
public double normalProbability(double x, Statistic s) {
// Distribution is symmetrical around mean
if(x < s.mean) {
double xp = 2*s.mean - x ;
return 1.0 - normalProbability(xp, s);
}
// How many standard deviations above the mean are we - scaled by 10X for table
double xs = 10*(x - s.mean) / s.stdev;
xs = Math.round(xs);
if(xs > 70) {
return 0.0;
}
return Q[(int)xs];
}
/**
* {@inheritDoc}
*/
@Override
public double compute(int[] activeColumns, int[] predictedColumns, double inputValue, long timestamp) {
if(inputValue == 0) {
throw new IllegalArgumentException("Selected anomaly mode Mode.LIKELIHOOD requires an \"inputValue\" to " +
"the compute() method.");
}
DateTime time = timestamp > 0 ? new DateTime(timestamp) : new DateTime();
// First compute raw anomaly score
double retVal = computeRawAnomalyScore(activeColumns, predictedColumns);
// low likelihood -> high anomaly
double probability = anomalyProbability(inputValue, retVal, time);
// Apply weighting if configured
retVal = isWeighted ? retVal * (1 - probability) : 1 - probability;
// Last, do moving-average if windowSize was specified
if(useMovingAverage) {
retVal = movingAverage.next(retVal);
}
return retVal;
}
/**
* Returns a flag indicating whether the specified params are valid.
* true if so, false if not
*
* @param params a {@link NamedTuple} containing { distribution, movingAverage, historicalLikelihoods }
* @return
*/
public boolean isValidEstimatorParams(NamedTuple params) {
if(params.get("distribution") == null || params.get("movingAverage") == null) {
return false;
}
Statistic stat = (Statistic)params.get("distribution");
if(stat.mean == 0 ||
stat.variance == 0 ||
stat.stdev == 0) {
return false;
}
return true;
}
/////////////////////////////////////////////////////////////////////////////
// AnomalyParams Class Definition //
/////////////////////////////////////////////////////////////////////////////
/**
*
* Extends the dictionary key lookup functionality of the parent class to add
* definite typing for parameter retrieval. Also handles output formatting of
* the contents to a serializable JSON format.
*
*
*
* {
* "distribution": # describes the distribution is-a {@link Statistic}
* {
* "name": STRING, # name of the distribution, such as 'normal'
* "mean": SCALAR, # mean of the distribution
* "variance": SCALAR, # variance of the distribution
*
* # There may also be some keys that are specific to the distribution
* },
*
* "historicalLikelihoods": [] # Contains the last windowSize likelihood
* # values returned
*
* "movingAverage": # stuff needed to compute a rolling average is-a {@link MovingAverage}
* # of the anomaly scores
* {
* "windowSize": SCALAR, # the size of the averaging window
* "historicalValues": [], # list with the last windowSize anomaly
* # scores
* "total": SCALAR, # the total of the values in historicalValues
* },
*
* }
*
*
*
* @author David Ray
*/
public static class AnomalyParams extends NamedTuple {
private static final long serialVersionUID = 1L;
private final Statistic distribution;
private final MovingAverage movingAverage;
private final double[] historicalLikelihoods;
private final int windowSize;
/**
* Constructs a new {@code AnomalyParams}
* @param keys
* @param values
*/
public AnomalyParams(String[] keys, Object... values) {
super(keys, values);
if(keys.length != 3 || values.length != 3) {
throw new IllegalArgumentException("AnomalyParams must have \"distribution\", \"movingAverage\", and \"historicalLikelihoods\"" +
" parameters. keys.length != 3 or values.length != 3");
}
this.distribution = (Statistic)get(KEY_DIST);
this.movingAverage = (MovingAverage)get(KEY_MVG_AVG);
this.historicalLikelihoods = (double[])get(KEY_HIST_LIKE);
this.windowSize = movingAverage.getWindowSize();
}
/**
* Returns the {@link Statistic} containing point calculations.
* @return
*/
public Statistic distribution() {
return distribution;
}
/**
* Returns the {@link MovingAverage} object
* @return
*/
public MovingAverage movingAverage() {
return movingAverage;
}
/**
* Returns the array of computed likelihoods
* @return
*/
public double[] historicalLikelihoods() {
return historicalLikelihoods;
}
/**
* Returns the window size of the moving average.
* @return
*/
public int windowSize() {
return windowSize;
}
/* (non-Javadoc)
* @see java.lang.Object#hashCode()
*/
@Override
public int hashCode() {
final int prime = 31;
int result = super.hashCode();
result = prime * result + ((distribution == null) ? 0 : distribution.hashCode());
result = prime * result + Arrays.hashCode(historicalLikelihoods);
result = prime * result + ((movingAverage == null) ? 0 : movingAverage.hashCode());
result = prime * result + windowSize;
return result;
}
/* (non-Javadoc)
* @see java.lang.Object#equals(java.lang.Object)
*/
@Override
public boolean equals(Object obj) {
if(this == obj)
return true;
if(!super.equals(obj))
return false;
if(getClass() != obj.getClass())
return false;
AnomalyParams other = (AnomalyParams)obj;
if(distribution == null) {
if(other.distribution != null)
return false;
} else if(!distribution.equals(other.distribution))
return false;
if(!Arrays.equals(historicalLikelihoods, other.historicalLikelihoods))
return false;
if(movingAverage == null) {
if(other.movingAverage != null)
return false;
} else if(!movingAverage.equals(other.movingAverage))
return false;
if(windowSize != other.windowSize)
return false;
return true;
}
}
// Table lookup for Q function, from wikipedia
// http://en.wikipedia.org/wiki/Q-function
private static double[] Q;
static {
Q = new double[71];
Q[0] = 0.500000000;
Q[1] = 0.460172163;
Q[2] = 0.420740291;
Q[3] = 0.382088578;
Q[4] = 0.344578258;
Q[5] = 0.308537539;
Q[6] = 0.274253118;
Q[7] = 0.241963652;
Q[8] = 0.211855399;
Q[9] = 0.184060125;
Q[10] = 0.158655254;
Q[11] = 0.135666061;
Q[12] = 0.115069670;
Q[13] = 0.096800485;
Q[14] = 0.080756659;
Q[15] = 0.066807201;
Q[16] = 0.054799292;
Q[17] = 0.044565463;
Q[18] = 0.035930319;
Q[19] = 0.028716560;
Q[20] = 0.022750132;
Q[21] = 0.017864421;
Q[22] = 0.013903448;
Q[23] = 0.010724110;
Q[24] = 0.008197536;
Q[25] = 0.006209665;
Q[26] = 0.004661188;
Q[27] = 0.003466974;
Q[28] = 0.002555130;
Q[29] = 0.001865813;
Q[30] = 0.001349898;
Q[31] = 0.000967603;
Q[32] = 0.000687138;
Q[33] = 0.000483424;
Q[34] = 0.000336929;
Q[35] = 0.000232629;
Q[36] = 0.000159109;
Q[37] = 0.000107800;
Q[38] = 0.000072348;
Q[39] = 0.000048096;
Q[40] = 0.000031671;
// From here on use the approximation in http://cnx.org/content/m11537/latest/
Q[41] = 0.000021771135897;
Q[42] = 0.000014034063752;
Q[43] = 0.000008961673661;
Q[44] = 0.000005668743475;
Q[45] = 0.000003551942468;
Q[46] = 0.000002204533058;
Q[47] = 0.000001355281953;
Q[48] = 0.000000825270644;
Q[49] = 0.000000497747091;
Q[50] = 0.000000297343903;
Q[51] = 0.000000175930101;
Q[52] = 0.000000103096834;
Q[53] = 0.000000059836778;
Q[54] = 0.000000034395590;
Q[55] = 0.000000019581382;
Q[56] = 0.000000011040394;
Q[57] = 0.000000006164833;
Q[58] = 0.000000003409172;
Q[59] = 0.000000001867079;
Q[60] = 0.000000001012647;
Q[61] = 0.000000000543915;
Q[62] = 0.000000000289320;
Q[63] = 0.000000000152404;
Q[64] = 0.000000000079502;
Q[65] = 0.000000000041070;
Q[66] = 0.000000000021010;
Q[67] = 0.000000000010644;
Q[68] = 0.000000000005340;
Q[69] = 0.000000000002653;
Q[70] = 0.000000000001305;
}
}
© 2015 - 2025 Weber Informatics LLC | Privacy Policy