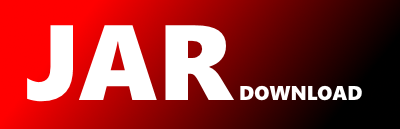
org.ojalgo.optimisation.convex.ActiveSetSolver Maven / Gradle / Ivy
/*
* Copyright 1997-2021 Optimatika
*
* Permission is hereby granted, free of charge, to any person obtaining a copy
* of this software and associated documentation files (the "Software"), to deal
* in the Software without restriction, including without limitation the rights
* to use, copy, modify, merge, publish, distribute, sublicense, and/or sell
* copies of the Software, and to permit persons to whom the Software is
* furnished to do so, subject to the following conditions:
*
* The above copyright notice and this permission notice shall be included in
* all copies or substantial portions of the Software.
*
* THE SOFTWARE IS PROVIDED "AS IS", WITHOUT WARRANTY OF ANY KIND, EXPRESS OR
* IMPLIED, INCLUDING BUT NOT LIMITED TO THE WARRANTIES OF MERCHANTABILITY,
* FITNESS FOR A PARTICULAR PURPOSE AND NONINFRINGEMENT. IN NO EVENT SHALL THE
* AUTHORS OR COPYRIGHT HOLDERS BE LIABLE FOR ANY CLAIM, DAMAGES OR OTHER
* LIABILITY, WHETHER IN AN ACTION OF CONTRACT, TORT OR OTHERWISE, ARISING FROM,
* OUT OF OR IN CONNECTION WITH THE SOFTWARE OR THE USE OR OTHER DEALINGS IN THE
* SOFTWARE.
*/
package org.ojalgo.optimisation.convex;
import static org.ojalgo.function.constant.PrimitiveMath.*;
import org.ojalgo.array.SparseArray;
import org.ojalgo.function.aggregator.Aggregator;
import org.ojalgo.function.aggregator.AggregatorFunction;
import org.ojalgo.function.aggregator.PrimitiveAggregator;
import org.ojalgo.matrix.store.MatrixStore;
import org.ojalgo.matrix.store.PhysicalStore;
import org.ojalgo.matrix.store.Primitive64Store;
import org.ojalgo.optimisation.GenericSolver;
import org.ojalgo.type.IndexSelector;
abstract class ActiveSetSolver extends ConstrainedSolver {
private static final String NEGATIVE_I_SLACK = "Negative I-slack! {}";
private static final String NONZERO_E_SLACK = "Nonzero E-slack! {}";
private final IndexSelector myActivator;
private int myConstraintToInclude = -1;
private MatrixStore myInvQC;
private final Primitive64Store myIterationX;
private boolean myShrinkSwitch = true;
private final Primitive64Store mySlackI;
ActiveSetSolver(final ConvexSolver.Builder matrices, final Options solverOptions) {
super(matrices, solverOptions);
int numberOfVariables = this.countVariables();
int numberOfEqualityConstraints = this.countEqualityConstraints();
int numberOfInequalityConstraints = this.countInequalityConstraints();
myActivator = new IndexSelector(numberOfInequalityConstraints);
myIterationX = Primitive64Store.FACTORY.make(numberOfVariables, 1L);
mySlackI = Primitive64Store.FACTORY.make(numberOfInequalityConstraints, 1L);
}
private void handleIterationSolution(final Primitive64Store iterX, final int[] excluded) {
// Subproblem solved successfully
final PhysicalStore soluX = this.getSolutionX();
iterX.modifyMatching(SUBTRACT, soluX);
final double normCurrentX = soluX.aggregateAll(Aggregator.LARGEST);
final double normStepX = iterX.aggregateAll(Aggregator.LARGEST);
if (this.isLogDebug()) {
this.log("Current: {} - {}", normCurrentX, soluX.asList());
this.log("Step: {} - {}", normStepX, iterX.asList());
}
if (this.isLogDebug() && options.validate) {
final PhysicalStore includedChange = this.getMatrixAI(this.getIncluded()).get().multiply(iterX).copy();
if (includedChange.count() > 0) {
this.log("Included-change: {}", includedChange.asList());
double introducedError = includedChange.aggregateAll(Aggregator.LARGEST);
if (!options.feasibility.isZero(introducedError)) {
this.log("Nonzero Included-change! {}", introducedError);
}
}
}
if (!options.solution.isSmall(normCurrentX, normStepX) && !ACCURACY.isSmall(normStepX, Math.max(normCurrentX, ONE))) {
// Non-zero && non-freak solution
double stepLength = ONE;
if (excluded.length > 0) {
final MatrixStore slack = this.getSlackI(excluded);
if (this.isLogDebug()) {
final MatrixStore change = this.getMatrixAI(excluded).get().multiply(iterX);
if (slack.count() != change.count()) {
throw new IllegalStateException();
}
final PhysicalStore steps = slack.copy();
steps.modifyMatching(DIVIDE, change);
this.log("Numer/slack: {}", slack.toRawCopy1D());
this.log("Denom/chang: {}", change.toRawCopy1D());
this.log("Looking for the largest possible step length (smallest positive scalar) among these: {}).", steps.toRawCopy1D());
}
for (int i = 0; i < excluded.length; i++) {
final SparseArray excludedInequalityRow = this.getMatrixAI(excluded[i]);
final double currentSlack = slack.doubleValue(i);
final double slackChange = excludedInequalityRow.dot(iterX);
final double fraction = Math.signum(currentSlack) == Math.signum(slackChange) && GenericSolver.ACCURACY.isSmall(slackChange, currentSlack)
? ZERO
: Math.abs(currentSlack) / slackChange;
// If the current slack is negative something has already gone wrong.
// Taking the abs value is to handle small negative values due to rounding errors
if (slackChange <= ZERO || GenericSolver.ACCURACY.isSmall(normStepX, slackChange)) {
// This constraint not affected
} else if (fraction >= ZERO) {
// Must check the step length
if (fraction < stepLength) {
stepLength = fraction;
this.setConstraintToInclude(excluded[i]);
if (this.isLogDebug()) {
this.log("Best so far: {} @ {} ({}).", stepLength, i, excluded[i]);
}
}
}
}
}
if (GenericSolver.ACCURACY.isZero(stepLength) && this.getConstraintToInclude() == this.getLastExcluded()) {
if (this.isLogProgress()) {
this.log("Break cycle on redundant constraints because step length {} on constraint {}", stepLength, this.getConstraintToInclude());
}
this.setConstraintToInclude(-1);
} else if (stepLength > ZERO) {
if (this.isLogProgress()) {
this.log("Performing update with step length {} adding constraint {}", stepLength, this.getConstraintToInclude());
}
iterX.axpy(stepLength, soluX);
} else if (this.isLogProgress()) {
this.log("Do nothing because step length {} and size {} but add constraint {}", stepLength, normStepX, this.getConstraintToInclude());
}
} else {
// Zero solution
if (this.isLogDebug()) {
this.log("Step too small (or freaky large)!");
}
this.setState(State.FEASIBLE);
}
if (this.isLogDebug()) {
this.log("Post iteration");
this.log("\tSolution: {}", soluX.asList());
this.log("\tL: {}", this.getSolutionL().asList());
}
if (this.isLogDebug() || options.validate) {
this.checkFeasibility();
}
}
private final void shrink() {
int toExclude = this.suggestConstraintToExclude();
if (toExclude < 0) {
if (myShrinkSwitch) {
toExclude = this.suggestUsingLagrangeMagnitude();
} else {
toExclude = this.suggestUsingVectorProjection();
}
myShrinkSwitch = !myShrinkSwitch;
}
if (this.isLogDebug()) {
this.log("Will remove {}", toExclude);
}
this.exclude(toExclude);
}
private final int suggestUsingLagrangeMagnitude() {
final int[] incl = myActivator.getIncluded();
final Primitive64Store soluL = this.getSolutionL();
final int numbEqus = this.countEqualityConstraints();
int toExclude = incl[0];
double maxWeight = ZERO;
for (int i = 0; i < incl.length; i++) {
final double value = soluL.doubleValue(numbEqus + incl[i]);
final double weight = ABS.invoke(value) * MAX.invoke(-value, ONE);
if (weight > maxWeight) {
maxWeight = weight;
toExclude = incl[i];
}
}
return toExclude;
}
private final int suggestUsingVectorProjection() {
final int[] incl = myActivator.getIncluded();
int lastIncluded = myActivator.getLastIncluded();
AggregatorFunction aggregator = PrimitiveAggregator.getSet().norm2();
SparseArray lastRow = this.getMatrixAI(lastIncluded);
lastRow.visitAll(aggregator);
double lastNorm = aggregator.doubleValue();
int toExclude = lastIncluded;
double maxWeight = ZERO;
// The weight is the absolute value of the cosine of the angle between the vectors (the constraint rows).
for (int i = 0; i < incl.length; i++) {
aggregator.reset();
SparseArray inclRow = this.getMatrixAI(incl[i]);
inclRow.visitAll(aggregator);
double inclNorm = aggregator.doubleValue();
final double weight = Math.abs(lastRow.dot(inclRow)) / lastNorm / inclNorm;
if (weight > maxWeight) {
maxWeight = weight;
toExclude = incl[i];
}
}
return toExclude;
}
protected int countExcluded() {
return myActivator.countExcluded();
}
protected int countIncluded() {
return myActivator.countIncluded();
}
protected void exclude(final int anIndexToExclude) {
myActivator.exclude(anIndexToExclude);
}
@Override
protected MatrixStore extractSolution() {
return super.extractSolution();
}
protected int[] getExcluded() {
return myActivator.getExcluded();
}
protected int[] getIncluded() {
return myActivator.getIncluded();
}
protected int getLastExcluded() {
return myActivator.getLastExcluded();
}
protected int getLastIncluded() {
return myActivator.getLastIncluded();
}
protected void include(final int anIndexToInclude) {
myActivator.include(anIndexToInclude);
}
@Override
protected final boolean initialise(final Result kickStarter) {
boolean ok = super.initialise(kickStarter);
myInvQC = this.getSolutionQ(this.getIterationC());
boolean feasible = false;
boolean usableKickStarter = kickStarter != null && kickStarter.getState().isApproximate();
if (usableKickStarter) {
this.getSolutionX().fillMatching(kickStarter);
if (kickStarter.getState().isFeasible()) {
feasible = true;
} else {
feasible = this.checkFeasibility();
}
}
if (!feasible) {
Result resultLP = this.solveLP();
if (feasible = resultLP.getState().isFeasible()) {
this.getSolutionX().fillMatching(resultLP);
this.getSolutionL().fillAll(ZERO);
}
}
if (feasible) {
this.setState(State.FEASIBLE);
this.resetActivator();
} else {
this.setState(State.INFEASIBLE);
this.getSolutionX().fillAll(ZERO);
}
if (this.isLogDebug()) {
this.log("Initial solution: {}", this.getSolutionX().copy().asList());
this.checkFeasibility();
}
return ok && this.getState().isFeasible();
}
@Override
protected final boolean needsAnotherIteration() {
if (this.isLogDebug()) {
this.log("\nNeedsAnotherIteration?");
}
int toInclude = -1;
int toExclude = -1;
if ((toInclude = this.suggestConstraintToInclude()) >= 0) {
if (this.isLogDebug()) {
this.log("Suggested to include: {}", toInclude);
}
myActivator.include(toInclude);
return true;
}
if ((toExclude = this.suggestConstraintToExclude()) >= 0) {
if (this.isLogDebug()) {
this.log("Suggested to exclude: {}", toExclude);
}
this.exclude(toExclude);
return true;
}
if (this.isLogDebug()) {
this.log("Stop!");
}
this.setState(State.OPTIMAL);
return false;
}
/**
* Find the minimum (largest negative) lagrange multiplier - for the active inequalities - to potentially
* deactivate.
*/
protected int suggestConstraintToExclude() {
int retVal = -1;
final int[] tmpIncluded = myActivator.getIncluded();
final int tmpLastIncluded = myActivator.getLastIncluded();
int tmpIndexOfLast = -1;
double tmpMin = POSITIVE_INFINITY;
double tmpVal;
// final MatrixStore tmpLI = this.getLI(tmpIncluded);
final MatrixStore tmpLI = this.getSolutionL().logical().offsets(this.countEqualityConstraints(), 0).row(tmpIncluded).get();
if (this.isLogDebug() && tmpLI.count() > 0L) {
this.log("Looking for the largest negative lagrange multiplier among these: {}.", tmpLI.copy().asList());
}
for (int i = 0; i < tmpLI.countRows(); i++) {
if (tmpIncluded[i] != tmpLastIncluded) {
tmpVal = tmpLI.doubleValue(i, 0);
if (tmpVal < ZERO && tmpVal < tmpMin && !GenericSolver.ACCURACY.isZero(tmpVal)) {
tmpMin = tmpVal;
retVal = i;
if (this.isLogDebug()) {
this.log("Best so far: {} @ {} ({}).", tmpMin, retVal, tmpIncluded[i]);
}
}
} else {
tmpIndexOfLast = i;
}
}
if (retVal < 0 && tmpIndexOfLast >= 0) {
tmpVal = tmpLI.doubleValue(tmpIndexOfLast, 0);
if (tmpVal < ZERO && tmpVal < tmpMin && !GenericSolver.ACCURACY.isZero(tmpVal)) {
tmpMin = tmpVal;
retVal = tmpIndexOfLast;
if (this.isLogProgress()) {
this.log("Only the last included needs to be excluded: {} @ {} ({}).", tmpMin, retVal, tmpIncluded[retVal]);
}
}
}
if (this.isLogProgress()) {
if (retVal < 0) {
this.log("Nothing to exclude");
} else {
this.log("Suggest to exclude: {} @ {} ({}).", tmpMin, retVal, tmpIncluded[retVal]);
}
}
return retVal >= 0 ? tmpIncluded[retVal] : retVal;
}
/**
* Find minimum (largest negative) slack - for the inactive inequalities - to potentially activate.
* Negative slack means the constraint is violated. Need to make sure it is enforced by activating it.
*/
protected int suggestConstraintToInclude() {
return this.getConstraintToInclude();
}
protected String toActivatorString() {
return myActivator.toString();
}
boolean checkFeasibility() {
boolean retVal = true;
PhysicalStore slackE = this.getSlackE();
PhysicalStore slackI = this.getSlackI();
if (retVal && slackE.count() > 0) {
if (this.isLogDebug()) {
this.log("E-slack: {}", slackE.asList());
}
double largestE = slackE.aggregateAll(Aggregator.LARGEST);
if (!options.feasibility.isZero(largestE)) {
retVal = false;
if (this.isLogDebug()) {
this.log(NONZERO_E_SLACK, largestE);
}
}
}
if (retVal && slackI.count() > 0) {
if (this.isLogDebug()) {
this.log("I-slack: {}", slackI.asList());
}
double minimumI = slackI.aggregateAll(Aggregator.MINIMUM);
if (minimumI < ZERO && !options.feasibility.isZero(minimumI)) {
retVal = false;
if (this.isLogDebug()) {
this.log(NEGATIVE_I_SLACK, minimumI);
}
}
}
return retVal;
}
@Override
final int countIterationConstraints() {
return this.countEqualityConstraints() + this.countIncluded();
}
int getConstraintToInclude() {
return myConstraintToInclude;
}
MatrixStore getInvQC() {
return myInvQC;
}
@Override
final MatrixStore getIterationA() {
final int numbEqus = this.countEqualityConstraints();
final int numbVars = this.countVariables();
final int[] incl = myActivator.getIncluded();
final PhysicalStore retVal = Primitive64Store.FACTORY.make(numbEqus + incl.length, numbVars);
if (numbEqus > 0) {
this.getMatrixAE().supplyTo(retVal.regionByLimits(numbEqus, numbVars));
}
for (int i = 0; i < incl.length; i++) {
this.getMatrixAI(incl[i]).supplyNonZerosTo(retVal.regionByRows(numbEqus + i));
}
return retVal;
}
@Override
final MatrixStore getIterationB() {
final int numbEqus = this.countEqualityConstraints();
final int[] incl = myActivator.getIncluded();
final PhysicalStore retVal = Primitive64Store.FACTORY.make(numbEqus + incl.length, 1);
for (int i = 0; i < numbEqus; i++) {
retVal.set(i, this.getMatrixBE().doubleValue(i));
}
for (int i = 0; i < incl.length; i++) {
retVal.set(numbEqus + i, this.getMatrixBI().doubleValue(incl[i]));
}
return retVal;
}
@Override
final MatrixStore getIterationC() {
// final MatrixStore tmpQ = this.getQ();
// final MatrixStore tmpC = this.getC();
//
// final PhysicalStore tmpX = this.getX();
//
// return tmpC.subtract(tmpQ.multiply(tmpX));
return this.getMatrixC();
}
Primitive64Store getIterationX() {
return myIterationX;
}
PhysicalStore getSlackI() {
MatrixStore mtrxBI = this.getMatrixBI();
PhysicalStore mtrxX = this.getSolutionX();
mySlackI.fillMatching(mtrxBI);
int nbInequalities = mtrxBI.getRowDim();
for (int i = 0; i < nbInequalities; i++) {
mySlackI.add(i, -this.getMatrixAI(i).dot(mtrxX));
}
return mySlackI;
}
MatrixStore getSlackI(final int[] rows) {
return this.getSlackI().logical().row(rows).get();
}
final void handleIterationResults(final boolean solved, final Primitive64Store iterX, final int[] included, final int[] excluded) {
this.incrementIterationsCount();
if (solved) {
this.handleIterationSolution(iterX, excluded);
} else if (this.isIterationAllowed()) {
// Assume Q solvable
// There must be a problem with the constraints
if (this.isLogProgress()) {
this.log("Constraints problem!");
}
if (included.length >= 1) {
// At least 1 active inequality
this.shrink();
this.performIteration();
} else {
// Should not be possible to end up here, infeasibility among
// the equality constraints should have been detected earlier.
this.setState(State.FAILED);
}
} else if (this.checkFeasibility()) {
// Feasible current solution
this.setState(State.FEASIBLE);
} else {
// Current solution somehow NOT feasible
this.setState(State.FAILED);
}
}
void resetActivator() {
myActivator.excludeAll();
int numbEqus = this.countEqualityConstraints();
int numbVars = this.countVariables();
int maxToInclude = numbVars - numbEqus;
if (this.isLogDebug() && numbEqus > numbVars) {
this.log("Redundant contraints!");
}
if (this.hasInequalityConstraints()) {
final MatrixStore inqSlack = this.getSlackI();
final int[] excl = this.getExcluded();
Primitive64Store lagrange = this.getSolutionL();
for (int i = 0; i < excl.length; i++) {
double slack = inqSlack.doubleValue(excl[i]);
if (ACCURACY.isZero(slack) && this.countIncluded() < maxToInclude) {
if (this.isLogDebug()) {
this.log("Will inlcude ineq {} with slack={}", i, slack);
}
this.include(excl[i]);
}
}
}
}
void setConstraintToInclude(final int constraintToInclude) {
myConstraintToInclude = constraintToInclude;
}
}
© 2015 - 2025 Weber Informatics LLC | Privacy Policy