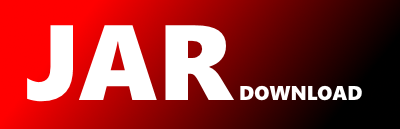
org.openimaj.feature.IntFV Maven / Gradle / Ivy
Go to download
Show more of this group Show more artifacts with this name
Show all versions of core-feature Show documentation
Show all versions of core-feature Show documentation
Core notion of features, usually denoted as arrays of data.
Definitions of features for all primitive types, features
with location and lists of features (both in memory and on disk).
/*
AUTOMATICALLY GENERATED BY jTemp FROM
/Users/jon/Work/openimaj/target/checkout/core/core-feature/src/main/jtemp/org/openimaj/feature/#T#FV.jtemp
*/
/**
* Copyright (c) 2011, The University of Southampton and the individual contributors.
* All rights reserved.
*
* Redistribution and use in source and binary forms, with or without modification,
* are permitted provided that the following conditions are met:
*
* * Redistributions of source code must retain the above copyright notice,
* this list of conditions and the following disclaimer.
*
* * Redistributions in binary form must reproduce the above copyright notice,
* this list of conditions and the following disclaimer in the documentation
* and/or other materials provided with the distribution.
*
* * Neither the name of the University of Southampton nor the names of its
* contributors may be used to endorse or promote products derived from this
* software without specific prior written permission.
*
* THIS SOFTWARE IS PROVIDED BY THE COPYRIGHT HOLDERS AND CONTRIBUTORS "AS IS" AND
* ANY EXPRESS OR IMPLIED WARRANTIES, INCLUDING, BUT NOT LIMITED TO, THE IMPLIED
* WARRANTIES OF MERCHANTABILITY AND FITNESS FOR A PARTICULAR PURPOSE ARE
* DISCLAIMED. IN NO EVENT SHALL THE COPYRIGHT OWNER OR CONTRIBUTORS BE LIABLE FOR
* ANY DIRECT, INDIRECT, INCIDENTAL, SPECIAL, EXEMPLARY, OR CONSEQUENTIAL DAMAGES
* (INCLUDING, BUT NOT LIMITED TO, PROCUREMENT OF SUBSTITUTE GOODS OR SERVICES;
* LOSS OF USE, DATA, OR PROFITS; OR BUSINESS INTERRUPTION) HOWEVER CAUSED AND ON
* ANY THEORY OF LIABILITY, WHETHER IN CONTRACT, STRICT LIABILITY, OR TORT
* (INCLUDING NEGLIGENCE OR OTHERWISE) ARISING IN ANY WAY OUT OF THE USE OF THIS
* SOFTWARE, EVEN IF ADVISED OF THE POSSIBILITY OF SUCH DAMAGE.
*/
package org.openimaj.feature;
import java.io.DataInput;
import java.io.DataOutput;
import java.io.IOException;
import java.io.PrintWriter;
import java.util.Arrays;
import java.util.List;
import java.util.Scanner;
import org.openimaj.util.concatenate.Concatenatable;
/**
* Basic int single-dimensional feature vector implementation
*
* @author Jonathon Hare
*/
public class IntFV extends ArrayFeatureVector implements Concatenatable, Cloneable {
private static final long serialVersionUID = 1L;
/**
* Construct an empty feature vector
*/
public IntFV() {}
/**
* Construct empty FV with given number of bins
* @param nbins the number of bins in each dimension
*/
public IntFV(int nbins) {
values = new int[nbins];
}
/**
* Construct from flattened values array and dimensions
* @param values the flat array of values
*/
public IntFV(int [] values) {
this.values = values;
}
/**
* Get the element at the given flat index
* @param x the flattened element index
* @return the value corresponding to x
*/
public int get(int x) {
return values[x];
}
/**
* Set the element at the given flat index
* @param value the value to set
* @param x the flattened element index
*/
void set(int value, int x) {
values[x] = value;
}
/**
* Element-wise normalisation to 0..1 using separated expected
* minimum and maximum values for each element of the underlying
* feature vector.
*
* @param min an array containing the minimum expected values
* @param max an array containing the maximum expected values
* @return feature vector with each value normalised to 0..1
*/
@Override
public DoubleFV normaliseFV(double [] min, double [] max) {
double [] dvals = asDoubleVector();
for (int i=0; i1) dvals[i] = 1;
}
return new DoubleFV(dvals);
}
/**
* Min-Max normalisation of the FV. Each element of the underlying
* feature vector is normalised to 0..1 based on the provided
* minimum and maximum expected values.
*
* @param min the minimum expected value
* @param max the maximum expected value
* @return feature vector with each value normalised to 0..1
*/
@Override
public DoubleFV normaliseFV(double min, double max) {
double [] dvals = asDoubleVector();
for (int i=0; i1) dvals[i] = 1;
}
return new DoubleFV(dvals);
}
/**
* Normalise the FV to unit area.
*
* @return feature vector with all elements summing to 1.
*/
@Override
public DoubleFV normaliseFV() {
double [] dvals = asDoubleVector();
double sum = 0;
for (int i=0; i ins) {
int l = values.length;
for (int i=0; i
© 2015 - 2024 Weber Informatics LLC | Privacy Policy