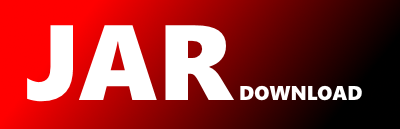
org.opensearch.search.aggregations.pipeline.SimulatedAnealingMinimizer Maven / Gradle / Ivy
Go to download
Show more of this group Show more artifacts with this name
Show all versions of opensearch Show documentation
Show all versions of opensearch Show documentation
OpenSearch subproject :server
/*
* SPDX-License-Identifier: Apache-2.0
*
* The OpenSearch Contributors require contributions made to
* this file be licensed under the Apache-2.0 license or a
* compatible open source license.
*/
/*
* Licensed to Elasticsearch under one or more contributor
* license agreements. See the NOTICE file distributed with
* this work for additional information regarding copyright
* ownership. Elasticsearch licenses this file to you under
* the Apache License, Version 2.0 (the "License"); you may
* not use this file except in compliance with the License.
* You may obtain a copy of the License at
*
* http://www.apache.org/licenses/LICENSE-2.0
*
* Unless required by applicable law or agreed to in writing,
* software distributed under the License is distributed on an
* "AS IS" BASIS, WITHOUT WARRANTIES OR CONDITIONS OF ANY
* KIND, either express or implied. See the License for the
* specific language governing permissions and limitations
* under the License.
*/
/*
* Modifications Copyright OpenSearch Contributors. See
* GitHub history for details.
*/
package org.opensearch.search.aggregations.pipeline;
import org.opensearch.common.collect.EvictingQueue;
/**
* A cost minimizer which will fit a MovAvgModel to the data.
*
* This optimizer uses naive simulated annealing. Random solutions in the problem space
* are generated, compared against the last period of data, and the least absolute deviation
* is recorded as a cost.
*
* If the new cost is better than the old cost, the new coefficients are chosen. If the new
* solution is worse, there is a temperature-dependent probability it will be randomly selected
* anyway. This allows the algo to sample the problem space widely. As iterations progress,
* the temperature decreases and the algorithm rejects poor solutions more regularly,
* theoretically honing in on a global minimum.
*/
public class SimulatedAnealingMinimizer {
/**
* Runs the simulated annealing algorithm and produces a model with new coefficients that, theoretically
* fit the data better and generalizes to future forecasts without overfitting.
*
* @param model The MovAvgModel to be optimized for
* @param train A training set provided to the model, which predictions will be
* generated from
* @param test A test set of data to compare the predictions against and derive
* a cost for the model
* @return A new, minimized model that (theoretically) better fits the data
*/
public static MovAvgModel minimize(MovAvgModel model, EvictingQueue train, double[] test) {
double temp = 1;
double minTemp = 0.0001;
int iterations = 100;
double alpha = 0.9;
MovAvgModel bestModel = model;
MovAvgModel oldModel = model;
double oldCost = cost(model, train, test);
double bestCost = oldCost;
while (temp > minTemp) {
for (int i = 0; i < iterations; i++) {
MovAvgModel newModel = oldModel.neighboringModel();
double newCost = cost(newModel, train, test);
double ap = acceptanceProbability(oldCost, newCost, temp);
if (ap > Math.random()) {
oldModel = newModel;
oldCost = newCost;
if (newCost < bestCost) {
bestCost = newCost;
bestModel = newModel;
}
}
}
temp *= alpha;
}
return bestModel;
}
/**
* If the new cost is better than old, return 1.0. Otherwise, return a double that increases
* as the two costs are closer to each other.
*
* @param oldCost Old model cost
* @param newCost New model cost
* @param temp Current annealing temperature
* @return The probability of accepting the new cost over the old
*/
private static double acceptanceProbability(double oldCost, double newCost, double temp) {
return newCost < oldCost ? 1.0 : Math.exp(-(newCost - oldCost) / temp);
}
/**
* Calculates the "cost" of a model. E.g. when run on the training data, how closely do the predictions
* match the test data
*
* Uses Least Absolute Differences to calculate error. Note that this is not scale free, but seems
* to work fairly well in practice
*
* @param model The MovAvgModel we are fitting
* @param train A training set of data given to the model, which will then generate predictions from
* @param test A test set of data to compare against the predictions
* @return A cost, or error, of the model
*/
private static double cost(MovAvgModel model, EvictingQueue train, double[] test) {
double error = 0;
double[] predictions = model.predict(train, test.length);
assert (predictions.length == test.length);
for (int i = 0; i < predictions.length; i++) {
error += Math.abs(test[i] - predictions[i]);
}
return error;
}
}
© 2015 - 2025 Weber Informatics LLC | Privacy Policy