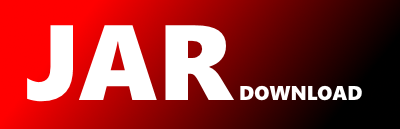
org.sonar.l10n.py.rules.python.S6918.html Maven / Gradle / Ivy
This rule raises an issue when a tensorflow.Variable
created inside of a tensorflow.function
is not a singleton.
Why is this an issue?
tensorflow.function
s only supports singleton tensorflow.Variable
s. This means the variable will be created on the first
call of the tensorflow.function
and will be reused across the subsequent calls. Creating a tensorflow.Variable
that is not a
singleton will raise a ValueError
.
import tensorflow as tf
@tf.function
def f(x):
v = tf.Variable(1.0)
return v
In the example above each time the function f
will be called a new tensorflow.Variable
will be created.
How to fix it
To fix this issue use the singleton pattern to avoid the creation of a tensorflow.Variable
multiple times.
Code examples
Noncompliant code example
import tensorflow as tf
class Count(tf.Module):
def __init__(self):
self.count = None
@tf.function
def __call__(self):
self.count = tf.Variable(0) # Noncompliant: the variable will be re-created for each call
return self.count.assign_add(1)
c = Count()
c() # ValueError is raised
c()
Compliant solution
import tensorflow as tf
class Count(tf.Module):
def __init__(self):
self.count = None
@tf.function
def __call__(self):
if self.count is None:
self.count = tf.Variable(0) # Compliant: the variable is created only on during the first call
return self.count.assign_add(1)
c = Count()
c()
c()
Resources
Documentation
- TensorFlow Documentation - Creating tf.Variables
© 2015 - 2024 Weber Informatics LLC | Privacy Policy