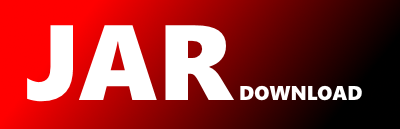
com.pulumi.gcp.diagflow.kotlin.outputs.CxFlowNluSettings.kt Maven / Gradle / Ivy
Go to download
Show more of this group Show more artifacts with this name
Show all versions of pulumi-gcp-kotlin Show documentation
Show all versions of pulumi-gcp-kotlin Show documentation
Build cloud applications and infrastructure by combining the safety and reliability of infrastructure as code with the power of the Kotlin programming language.
@file:Suppress("NAME_SHADOWING", "DEPRECATION")
package com.pulumi.gcp.diagflow.kotlin.outputs
import kotlin.Double
import kotlin.String
import kotlin.Suppress
/**
*
* @property classificationThreshold To filter out false positive results and still get variety in matched natural language inputs for your agent, you can tune the machine learning classification threshold.
* If the returned score value is less than the threshold value, then a no-match event will be triggered. The score values range from 0.0 (completely uncertain) to 1.0 (completely certain). If set to 0.0, the default of 0.3 is used.
* @property modelTrainingMode Indicates NLU model training mode.
* * MODEL_TRAINING_MODE_AUTOMATIC: NLU model training is automatically triggered when a flow gets modified. User can also manually trigger model training in this mode.
* * MODEL_TRAINING_MODE_MANUAL: User needs to manually trigger NLU model training. Best for large flows whose models take long time to train.
* Possible values are: `MODEL_TRAINING_MODE_AUTOMATIC`, `MODEL_TRAINING_MODE_MANUAL`.
* @property modelType Indicates the type of NLU model.
* * MODEL_TYPE_STANDARD: Use standard NLU model.
* * MODEL_TYPE_ADVANCED: Use advanced NLU model.
* Possible values are: `MODEL_TYPE_STANDARD`, `MODEL_TYPE_ADVANCED`.
*/
public data class CxFlowNluSettings(
public val classificationThreshold: Double? = null,
public val modelTrainingMode: String? = null,
public val modelType: String? = null,
) {
public companion object {
public fun toKotlin(javaType: com.pulumi.gcp.diagflow.outputs.CxFlowNluSettings): CxFlowNluSettings = CxFlowNluSettings(
classificationThreshold = javaType.classificationThreshold().map({ args0 -> args0 }).orElse(null),
modelTrainingMode = javaType.modelTrainingMode().map({ args0 -> args0 }).orElse(null),
modelType = javaType.modelType().map({ args0 -> args0 }).orElse(null),
)
}
}
© 2015 - 2024 Weber Informatics LLC | Privacy Policy