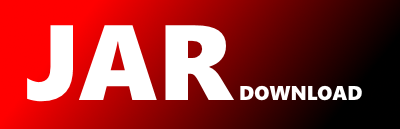
org.apache.spark.ml.odkl.NullToNaNVectorAssembler.scala Maven / Gradle / Ivy
The newest version!
package org.apache.spark.ml.odkl
import org.apache.spark.ml.feature.VectorAssembler
import org.apache.spark.ml.param.shared.HasInputCols
import org.apache.spark.ml.param.shared.HasOutputCol
import org.apache.spark.ml.util.DefaultParamsReadable
import org.apache.spark.ml.util.DefaultParamsWritable
import org.apache.spark.ml.util.Identifiable
import org.apache.spark.sql.types.BooleanType
import org.apache.spark.sql.types.DoubleType
import org.apache.spark.sql.types.NumericType
import org.apache.spark.sql.types.StructField
import org.apache.spark.sql.types.StructType
import org.apache.spark.SparkException
import org.apache.spark.annotation.{Since, Experimental}
import org.apache.spark.ml.Transformer
import org.apache.spark.ml.attribute.{Attribute, AttributeGroup, NumericAttribute, UnresolvedAttribute}
import org.apache.spark.ml.param.ParamMap
import org.apache.spark.mllib.linalg.{Vector, VectorUDT, Vectors}
import org.apache.spark.sql.{DataFrame, Row}
import org.apache.spark.sql.functions
/**
* :: Experimental ::
* A feature transformer that merges multiple columns into a vector column.
*
* This class is a copy of VectorAssembler with two enhancements: support for nulls (replaced to
* NaNs) and pattern matching extracted from the inner loop.
*/
@Experimental
class NullToNaNVectorAssembler(override val uid: String)
extends Transformer with HasInputCols with HasOutputCol with HasColumnAttributeMap
with DefaultParamsWritable {
def this() = this(Identifiable.randomUID("nullToNanVecAssembler"))
/** @group setParam */
def setInputCols(value: Array[String]): this.type = set(inputCols, value)
/** @group setParam */
def setOutputCol(value: String): this.type = set(outputCol, value)
override def transform(dataset: DataFrame): DataFrame = {
// Schema transformation.
val schema = dataset.schema
lazy val first = dataset.first()
// Analyze fields metadata if available
val prepared: Array[(StructField, Array[Attribute])] = $(inputCols).map { c =>
val field = schema(c)
val index = schema.fieldIndex(c)
val attributeName = getColumnAttributeName(c)
field -> (field.dataType match {
case DoubleType =>
val attr = Attribute.fromStructField(field)
// If the input column doesn't have ML attribute, assume numeric.
if (attr == UnresolvedAttribute) {
Array[Attribute](NumericAttribute.defaultAttr.withName(attributeName))
} else {
Array[Attribute](attr.withName(attributeName))
}
case _: NumericType | BooleanType =>
// If the input column type is a compatible scalar type, assume numeric.
Array[Attribute](NumericAttribute.defaultAttr.withName(attributeName))
case _: VectorUDT =>
val group = AttributeGroup.fromStructField(field)
if (group.attributes.isDefined) {
// If attributes are defined, copy them with updated names.
group.attributes.get.map { attr =>
if (attr.name.isDefined) {
// TODO: Define a rigorous naming scheme.
attr.withName(attributeName + "_" + attr.name.get)
} else {
attr
}
}
} else {
// Otherwise, treat all attributes as numeric. If we cannot get the number of attributes
// from metadata, check the first row.
val numAttrs = group.numAttributes.getOrElse(first.getAs[Vector](index).size)
Array.tabulate(numAttrs){ i => NumericAttribute.defaultAttr.withName(s"${attributeName}_$i").asInstanceOf[Attribute] }
}
case otherType =>
throw new SparkException(s"VectorAssembler does not support the $otherType type")
})
}
// Create overall metadata
val attrs: Array[Attribute] = prepared.flatMap(_._2)
val metadata = new AttributeGroup($(outputCol), attrs).toMetadata()
// Construct assembler functions for each part
val assemblers : Array[(Any,Int,Array[Double]) => Int] = prepared.map { x =>
val field = x._1
val attributes = x._2
field.dataType match {
case _: NumericType =>
(value : Any, start: Int, data: Array[Double]) => {
if(value == null) {
data(start) = Double.NaN
} else {
data(start) = value.asInstanceOf[Number].doubleValue()
}
1
}
case _: BooleanType =>
(value : Any, start: Int, data: Array[Double]) => {
if(value == null) {
data(start) = Double.NaN
} else {
data(start) = if (value.asInstanceOf[Boolean]) 1.0 else 0.0
}
1
}
case _: VectorUDT =>
(value : Any, start: Int, data: Array[Double]) => {
if(value == null) {
for(i <- x._2.indices) {
data(start + i) = Double.NaN
}
} else {
require(value.asInstanceOf[Vector].size == x._2.length, s"All vectors in field ${field.name} expected to be of size ${x._2.length}")
value.asInstanceOf[Vector].foreachActive((i, v) => data(start + i) = v)
}
x._2.length
}
case otherType =>
throw new SparkException(s"VectorAssembler does not support the $otherType type")
}
}
// Create the overal transformation function
val assembleFunc = functions.udf { r: Row =>
val data = new Array[Double](attrs.length)
var start = 0
for(i <- 0 until r.size) {
start = start + assemblers(i)(r.get(i), start, data)
}
Vectors.dense(data).compressed
}
// Apply the function
dataset.withColumn(
$(outputCol),
assembleFunc(functions.struct($(inputCols).map(c => dataset(c)): _*)),
metadata)
}
override def transformSchema(schema: StructType): StructType = {
val inputColNames = $(inputCols)
val outputColName = $(outputCol)
val inputDataTypes = inputColNames.map(name => schema(name).dataType)
inputDataTypes.foreach {
case _: NumericType | BooleanType =>
case t if t.isInstanceOf[VectorUDT] =>
case other =>
throw new IllegalArgumentException(s"Data type $other is not supported.")
}
if (schema.fieldNames.contains(outputColName)) {
throw new IllegalArgumentException(s"Output column $outputColName already exists.")
}
StructType(schema.fields :+ new StructField(outputColName, new VectorUDT, true))
}
override def copy(extra: ParamMap): NullToNaNVectorAssembler = defaultCopy(extra)
}
@Since("1.6.0")
object NullToNaNVectorAssembler extends DefaultParamsReadable[NullToNaNVectorAssembler]
© 2015 - 2025 Weber Informatics LLC | Privacy Policy