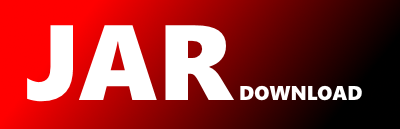
resources.wrappers.FileJsonKeras.gate-lf-python-data.gatelfdata.features.py Maven / Gradle / Ivy
Go to download
Show more of this group Show more artifacts with this name
Show all versions of learningframework Show documentation
Show all versions of learningframework Show documentation
A GATE plugin that provides many different machine learning
algorithms for a wide range of NLP-related machine learning tasks like
text classification, tagging, or chunking.
"""Module for the Features class"""
import sys
import logging
from gatelfdata.featurenumeric import FeatureNumeric
from gatelfdata.featurenominalembs import FeatureNominalEmbs
from gatelfdata.featureboolean import FeatureBoolean
from gatelfdata.featurengram import FeatureNgram
logger = logging.getLogger(__name__)
logger.setLevel(logging.INFO)
streamhandler = logging.StreamHandler(stream=sys.stderr)
formatter = logging.Formatter(
'%(asctime)s %(name)-12s %(levelname)-8s %(message)s')
streamhandler.setFormatter(formatter)
logger.addHandler(streamhandler)
class Features(object):
def make_feature(self, fname, datatype, attribute, featurestats, vocabs):
"""Helper function to create a specific feature gets called as part of __init__"""
kind = attribute["featureCode"]
logger.debug("Making feature for kind/name/type/attr: %r/%r/%r/%r", kind, fname, datatype, attribute)
if kind == "N":
# create an ngram feature, based on a simple feature of type nominal
ret = FeatureNgram(fname, attribute, featurestats, vocabs.get_vocab(attribute))
else:
# create a simple feature of the correct type
if datatype == "nominal":
# create a nominal feature, represented through embeddings or onehot
# We represent both by featurenominalembs, both get converted into a value
# index initiallly. However for onehot, the embedding vectors are just the onehot
# vectors (except for padding which is still an all-zero vector).
logger.debug("About to create feature, vocabs is %s" % (vocabs,))
ret = FeatureNominalEmbs(fname, attribute, featurestats, vocabs.get_vocab(attribute))
elif datatype == "numeric":
# simple numeric feature
ret = FeatureNumeric(fname, attribute, featurestats)
elif datatype == "boolean":
# simple boolean feature
ret = FeatureBoolean(fname, attribute, featurestats)
else:
raise Exception("Odd datatype: ", datatype)
logger.debug("Returning: %r", ret)
return ret
def __init__(self, meta, vocabs):
# initialisation consists of going through the meta info and
# creating all the individual feature instances and storing them
# in here in a list.
# NOTE: we should go through the actual features, not the attributes, so we do
# not really need anything that represents an attributelist since this is
# just a fixed number of simple attributes.
# meta: either a string or the meta information already read in and parsed.
self.meta = meta
self.vocabs = vocabs
self.isSequence = meta["isSequence"]
if self.isSequence:
self.seq_max = meta["sequLengths.max"]
self.seq_avg = meta["sequLengths.mean"]
# now we have the meta, create the list of features
self.features = []
attrs = self.meta["featureInfo"]["attributes"]
stats = self.meta["featureStats"]
# The LF metadata is per feature, not per embedding type of the feature, so
# we first need to combine the counts per feature for each of the types here.
for f in self.meta["features"]:
dt = f["datatype"]
attrnr = f["attrid"]
attrinfo = attrs[attrnr]
# attrcode = attrinfo.get("code")
if dt == "nominal":
self.vocabs.setup_vocab(attrinfo, stats[f["name"]])
self.vocabs.finish()
for f in self.meta["features"]:
dt = f["datatype"]
attrnr = f["attrid"]
fname = f["name"]
# attrkind = f["kind"]
# get a bit more info from the corresponding attribute metadata
attrinfo = attrs[attrnr]
fstats = stats[fname]
thefeature = self.make_feature(fname, dt, attrinfo, fstats, self.vocabs)
logger.debug("Features: appending feature=%r", thefeature)
self.features.append(thefeature)
def _convert_featurevec(self, valuelist, idxs=None, normalize=None):
if not idxs and (len(valuelist) != len(self.features)):
raise Exception("Wrong number of values passed, expected", len(self.features), "got", len(valuelist))
if idxs and len(idxs) > len(valuelist):
raise Exception("Wrong number of idxs passed, got", len(idxs), "but got values:", len(valuelist))
if idxs and len(idxs) > len(self.features):
raise Exception("Wrong number of idxs passed, got", len(idxs), "but got features:", len(self.features))
if idxs:
valuelist = [valuelist[i] for i in idxs]
features = [self.features[i] for i in idxs]
else:
features = self.features
values = []
for i in range(len(features)):
res = features[i](valuelist[i], normalize=normalize)
values.append(res)
return values
def __iter__(self):
return iter(self.features)
def __getitem__(self, item):
return self.features[item]
def __call__(self, valuelist, idxs=None, normalize=None):
# For a feature vector:
# this will go through each input and run it through the stored feature
# instance, and the values will get put into the result list and returned
# Note that for ngram attributes, the "value" to put into the list is itself a list
# (of embedding indices).
# For a sequence of feature vectors: each feature vector gets converted
# in the normal way, targets as well
# NOTE: not sure yet how to handle nominals that are onehot encoded! In some cases
# we want to instances in some we want the vectors .. see featurenominal1ofk
if self.isSequence:
out_indep = []
for fv in valuelist:
out_indep.append(self._convert_featurevec(fv, idxs=idxs))
return out_indep
else:
values = self._convert_featurevec(valuelist, idxs=idxs)
return values
def __call__OLD(self, valuelist, idxs=None):
# For a feature vector:
# this will go through each input and run it through the stored feature
# instance, and the values will get put into the result list and returned
# Note that for ngram attributes, the "value" to put into the list is itself a list
# (of embedding indices).
# For a sequence of feature vectors: will return a list/vector
# for each feature where each element corresponds to a sequence element
# So the representation gets changed from a list of feature vectors
# of values to a list of values for each feature
if self.isSequence:
# for now we do this in an easy to understand but maybe slow way:
# first go convert each of the feature vectors in the sequence
# then convert the resulting list of lists
seqofvecs = []
for el in valuelist:
vals4featurevec = self._convert_featurevec(el, idxs=idxs)
seqofvecs.append(vals4featurevec)
# now each element in sequofvecs should have as many elements
# as there are features, just transpose that matrix
return [l for l in map(list, zip(*seqofvecs))]
else:
values = self._convert_featurevec(valuelist, idxs=idxs)
return values
def size(self):
return len(self.features)
def __repr__(self):
fl = [f.__repr__() for f in self.features]
return "Features(features=%r)" % fl
def __str__(self):
fl = [f.__str__() for f in self.features]
return "Features("+",".join(fl)+")"
def pretty_print(self, file=sys.stdout):
print("Features:", file=file)
for f in self.features:
print(" ", f, file=file)
© 2015 - 2025 Weber Informatics LLC | Privacy Policy