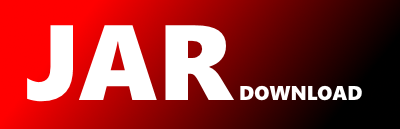
resources.wrappers.FileJsonPyTorch.gate-lf-python-data.docs.pythondoc.gatelfdata.dataset.html Maven / Gradle / Ivy
Show all versions of learningframework Show documentation
gatelfdata.dataset module — GATE LF Python-Data documentation
gatelfdata.dataset module¶
Module for the Dataset class
-
class
gatelfdata.dataset.
Dataset
(metafile, reuse_files=False, config=None, targets_need_padding=False)[source]¶
Bases: object
Class representing training data present in the meta and data files.
After creating the Dataset instance, the attribute .meta contains the loaded metadata.
Then, the instances_as_string and instances_as_data methods can be used to return an
iterable.
-
batches_converted
(train=True, file=None, reshape=True, convert=False, batch_size=100, as_numpy=False, pad_left=False)[source]¶
Return a batch of instances for training. If reshape is True, this reshapes the data in the following ways:
For classification, the independent part is a list of batchsize values for each feature. So for
a batch size of 100 and 18 features, the inputs are a list of 18 lists of 100 values each.
If the feature itself is a sequence (i.e. comes from an ngram), then the list corresponding
to that feature contains 100 lists.
For sequence tagging, the independent part is a list of features, where each of the per-feature lists
contains 100 (batch size) elements, and each of these elements is a list with as many elements
as the corresponding sequence contains.
If reshape is True (the default), then the batch gets reshaped using the reshape_batch method.
-
batches_original
(train=True, file=None, reshape=True, batch_size=100, pad_left=False, as_numpy=False)[source]¶
Return a batch of instances in original format for training.
-
convert_dep
(dep, is_batch=False, as_onehot=False)[source]¶
Convert the dependent part of an original representation into the converted representation
where strings are replaced by one hot vectors.
If as_onehot is True, then nominal targets is onverted to onehot float vectors instead of
integer indices (ignored for other target types).
-
convert_indep
(indep, normalize=None)[source]¶
Convert the independent part of an original representation into the converted representation
where strings are replaced by word indices or one hot vectors.
If normalize is None then the normalization will be performed according to the default
for the feature, otherwise it should be one of “minmax”, “meanvar”, or False or a normalizing function.
If False, normalization is turned off explicitly, otherwise the normalization function is used.
This parameter is ignored for all features which are not numeric.
-
convert_instance
(instance, normalize=’meanvar’, is_reshaped_batch=False)[source]¶
Convert an original representation of an instance as read from json to the converted representation.
This will also by default automatically normalize all numeric features, this can be changed by setting
the normalize parameter (see convert_indep).
If is_reshaped_batch is True, then we expect a batch of reshaped instances instead of
a single instance
Note: if the instance is a string, it is assumed it is still in json format and will get converted first.
-
convert_to_file
(outfile=None, infile=None)[source]¶
Copy the whole data file (or if infile is not None, that file) to a converted version.
The default file name is used if outfile is None, otherwise the file specified is used.
-
static
data4meta
(metafilename)[source]¶
Given the path to a meta file, return the path to a data file
-
feature_types_converted
()[source]¶
Returns a list with the converted types of the features as a string name.
Possible values are ‘float’, ‘index’, ‘indexlist’
-
feature_types_original
()[source]¶
Returns a list with the original types of the features as a string name.
Possible values are ‘nominal’, ‘number’, ‘ngram’, ‘boolean’
-
get_index_feature_idxs
()[source]¶
Return a list of indices of all nominal features represented by some index and
ultimately by a vector
-
get_index_features
()[source]¶
Return a list of all nominal features represented by some index and
ultimately by a vector
-
get_indexlist_feature_idxs
()[source]¶
Return a list of indices for all features which are ngrams, i.e. lists of embs.
-
get_info
()[source]¶
Return a concise description of the learning problem that makes it easier to understand
what is going on and what kind of network needs to get created.
-
instances_as_string
(train=False, file=None)[source]¶
Returns an iterable that allows to read the original instance data rows as a single string.
That string can be converted into the actual original representation by parsing it as json.
If train is set to True, then instead of the original data file, the train file created
with the split() method is used. If file is not None, train is ignored and the file specified
is read instead.
-
instances_converted
(train=True, file=None, convert=False)[source]¶
This reads instances and returns them in converted format. The instances are either
read from a file in original format and converted on the fly (convert=True) or from a file
that has already been converted as e.g. created with the split() or copy_to_converted() methods.
If the file parameter is not None, then that file is read, otherwise if the train parameter is
False then the original data file is read, otherwise if the train parameter is True, the
train file is read.
-
instances_original
(train=False, file=None)[source]¶
Returns an iterable that allows to read the instances from a file in original format.
This file is the original data file by default, but could also the train file created with
the split() method or any other file derived from the original data file.
-
static
load_meta
(metafile)[source]¶
Static method for just reading and returning the meta data for this dataset.
-
modified4meta
(name_part=None, dirname=None)[source]¶
Helper method to construct the full path of one of the files this class creates from
the original meta/data files. If dir is given, then it is the containing directory for the file.
The name_part parameter specifies the part in the file name that will replace the “meta”
in the original metafilename.
-
static
pad_list_
(thelist, tosize, pad_left=False, pad_value=None)[source]¶
Pads the list to have size elements, inserting the pad_value as needed,
left or right depending on pad_left. CAUTION! Modifies thelist and also returns it.
-
static
pad_matrix_
(matrix, tosize=None, pad_left=False, pad_value=None)[source]¶
Given a list of lists, pads all the inner list to size length or if size is None, first determines
the longest inner list and pads to that length. CAUTION: modifies the matrix!
-
reshape_batch
(instances, as_numpy=False, pad_left=False, from_original=False, pad=True, indep_only=False)[source]¶
Reshape the list of converted instances into what is expected for training on a batch.
NOTE: for non-sequence instances, we pad all list-typed features to the maximum length. If from_original
is true, the padding is done with empty strings, otherwise with integer zeros.
NOTE: as_numpy=True for from_original=True currently only converts the result of converting
the outermost list to a numpy array which will automatically also convert the embedded lists.
-
static
reshape_batch_helper
(instances, as_numpy=False, pad_left=False, from_original=False, pad=True, n_features=None, is_sequence=None, feature_types=None, target=None, indep_only=False)[source]¶
Reshapes a list of instances where each instance is a two-element list of an independent and dependent/target
part into a tuple where the first part is a list of features and the second part is the list of targets.
If the instances are not for sequence tagging, then each list that corresponds to a feature contains as many
values as there are instances. If the value of the feature is a sequence, then each value is a padded list.
For sequence tagging, The independent part contains as many lists as there are features, each of these
lists contains as many elements as there are instances. These elements in turn are lists, representing the
values of the feature for each feature vector in the sequence for the instance.
The feature_types list must be specified if is_sequence is True, in that case, n_features is not needed.
If indep_only is True, this will not expect targets and only reshape the independent features.
IMPORTANT: this pads all independent features based on their type, with indices getting padded using 0
and all dependent indices using -1!!! If target is specified, the targets are represented by a
onehot vector instead.
-
split
(outdir=None, validation_size=None, validation_part=0.1, random_seed=1, convert=False, keep_orig=False, reuse_files=False, validation_file=None)[source]¶
This splits the original file into an actual training file and a validation set file.
This creates two new files in the same location as the original files, with the “data”/”meta”
parts of the name replaced with “val” for validation and “train” for training. If converted is
set to True, then instead of the original data, the converted data is getting split and
saved, in that case the name parts are “converted.var” and “coverted.train”. If keep_orig is
set to True, then both the original and the converted format files are created. Depending on which
format files are created, subsequent calls to the batches_converted or batches_orig can be made.
If outdir is specified, the files will get stored in that directory instead of the directory
where the meta/data files are stored.
If random_seed is set to 0 or None, the random seed generator does not get initialized.
If reuse_files is True and the files that would have been created are already there
the method does nothing for that file, assuming, but not checking that the contents is correct.
If validation_file is not none, then validation_size and validation_part are ignored and
the whole original file is used as training file, the given validation_file is expected to
be a data file that fits the meta, and the content of the validation file is used.
-
validation_set_converted
(as_numpy=False, as_batch=False)[source]¶
Read and return the validation set instances in converted format, optionally converted to
batch format and if in batch format, optionally with numpy arrays. Fir this to work the split()
method must have been run before with convert set to True.