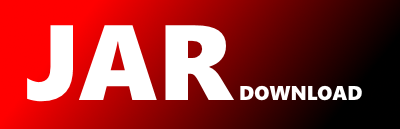
gate.util.ClassificationMeasures Maven / Gradle / Ivy
Go to download
GATE - general achitecture for text engineering - is
open source software capable of solving almost any text processing problem.
This artifact enables you to embed the core GATE Embedded with its essential dependencies.
You will able to use the GATE Embedded API and load and store GATE XML documents. This
artifact is the perfect dependency for CREOLE plugins or for applications that need to customize
the GATE dependencies due to confict with their own dependencies or for lower footprint.
The newest version!
Please wait ...