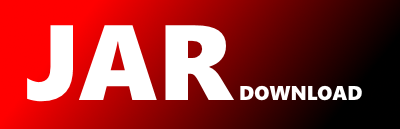
Download JAR files tagged by algorithm with all dependencies
mrglvq from group de.cit-ec.ml (version 0.1.0)
This project contains a Java implementation of median relational generalized learning vector
quantization as proposed by Nebel, Hammer, Frohberg, and Villmann
(2015, doi:10.1016/j.neucom.2014.12.096). Given a matrix of pairwise distances D and a
vector of labels Y it identifies prototypical data points (i.e. rows of D) which help
to classify the data set using a simple nearest neighbor rule. In particular, the algorithm
optimizes the generalized learning vector quantization cost function (Sato and Yamada, 1995)
via an expectation maximization scheme where in each iteration one prototype 'jumps' to
another data point in order to improve the cost function. If the cost function can not be
improved anymore for any of the data points, the algorithm terminates.
Artifact mrglvq
Group de.cit-ec.ml
Version 0.1.0
Last update 27. January 2018
Organization not specified
URL https://gitlab.ub.uni-bielefeld.de/bpaassen/median_relational_glvq
License The GNU General Public License, Version 3
Dependencies amount 1
Dependencies rng,
There are maybe transitive dependencies!
Group de.cit-ec.ml
Version 0.1.0
Last update 27. January 2018
Organization not specified
URL https://gitlab.ub.uni-bielefeld.de/bpaassen/median_relational_glvq
License The GNU General Public License, Version 3
Dependencies amount 1
Dependencies rng,
There are maybe transitive dependencies!
torodb-parent from group com.torodb (version 0.40)
ToroDB is an open source project that turns your RDBMS into a
MongoDB-compatible server, supporting the MongoDB query API and
MongoDB's replication, but storing your data into a reliable and trusted
ACID database. ToroDB currently supports PostgreSQL as a backend, but
others will be added in the future.
ToroDB natively implements the MongoDB protocol, so you can use it with
MongoDB tools and drivers, and features a document-to-relational mapping
algorithm that transforms the JSON documents into relational tables.
ToroDB also offers a native SQL layer and automatic data normalization
and partitioning based on JSON documents' implicit schema.
Group: com.torodb Artifact: torodb-parent
Show all versions
Show all versions
There is no JAR file uploaded. A download is not possible! Please choose another version.
0 downloads
Artifact torodb-parent
Group com.torodb
Version 0.40
Last update 11. June 2016
Organization 8Kdata
URL http://www.torodb.com
License GNU AFFERO GENERAL PUBLIC LICENSE, Version 3, 19 November 2007
Dependencies amount 0
Dependencies No dependencies
There are maybe transitive dependencies!
Group com.torodb
Version 0.40
Last update 11. June 2016
Organization 8Kdata
URL http://www.torodb.com
License GNU AFFERO GENERAL PUBLIC LICENSE, Version 3, 19 November 2007
Dependencies amount 0
Dependencies No dependencies
There are maybe transitive dependencies!
ijp-toolkit_2.12.0-M4 from group net.sf.ij-plugins (version 2.1.0)
<html>IJ Plugins Toolkit is a set of ImageJ plugins grouped into:<ul> <li>3D IO - import and export of data in 3D formats.</li> <li>3D Toolkit - operations on stacks interpreted as 3D images, including morphological operations.</li> <li>Color - color space conversion, color edge detection (color and multi-band images).</li> <li>Filters - fast median filters and various anisotropic diffusion filters.</li> <li>Graphics - Texture Synthesis - A plugin to perform texture synthesis using the image quilting algorithm of Efros and Freeman.</li> <li>Segmentation - image segmentation through clustering, thresholding, and region growing.</li></ul></html>
Artifact ijp-toolkit_2.12.0-M4
Group net.sf.ij-plugins
Version 2.1.0
Last update 09. May 2016
Organization net.sf.ij-plugins
URL https://ij-plugins.sf.net
License LGPL-2.1
Dependencies amount 4
Dependencies scala-library, commons-math3, jgoodies-binding, ij,
There are maybe transitive dependencies!
Group net.sf.ij-plugins
Version 2.1.0
Last update 09. May 2016
Organization net.sf.ij-plugins
URL https://ij-plugins.sf.net
License LGPL-2.1
Dependencies amount 4
Dependencies scala-library, commons-math3, jgoodies-binding, ij,
There are maybe transitive dependencies!
RBFNetwork from group nz.ac.waikato.cms.weka (version 1.0.8)
RBFNetwork implements a normalized Gaussian radial basisbasis function network.
It uses the k-means clustering algorithm to provide the basis functions and learns either a logistic regression (discrete class problems) or linear regression (numeric class problems) on top of that. Symmetric multivariate Gaussians are fit to the data from each cluster. If the class is nominal it uses the given number of clusters per class. RBFRegressor implements radial basis function networks for regression, trained in a fully supervised manner using WEKA's Optimization class by minimizing squared error with the BFGS method. It is possible to use conjugate gradient descent rather than BFGS updates, which is faster for cases with many parameters, and to use normalized basis functions instead of unnormalized ones.
11 downloads
Artifact RBFNetwork
Group nz.ac.waikato.cms.weka
Version 1.0.8
Last update 16. January 2015
Organization University of Waikato, Hamilton, NZ
URL http://weka.sourceforge.net/doc.packages/RBFNetwork
License GNU General Public License 3
Dependencies amount 1
Dependencies weka-dev,
There are maybe transitive dependencies!
Group nz.ac.waikato.cms.weka
Version 1.0.8
Last update 16. January 2015
Organization University of Waikato, Hamilton, NZ
URL http://weka.sourceforge.net/doc.packages/RBFNetwork
License GNU General Public License 3
Dependencies amount 1
Dependencies weka-dev,
There are maybe transitive dependencies!
DTNB from group nz.ac.waikato.cms.weka (version 1.0.3)
0 downloads
localOutlierFactor from group nz.ac.waikato.cms.weka (version 1.0.4)
A filter that applies the LOF (Local Outlier Factor) algorithm to compute an outlier score for each instance in the data. Can use multiple cores/cpus to speed up the LOF computation for large datasets. Nearest neighbor search methods and distance functions are pluggable.
For more information, see:
Markus M. Breunig, Hans-Peter Kriegel, Raymond T. Ng, Jorg Sander (2000). LOF: Identifying Density-Based Local Outliers. ACM SIGMOD Record. 29(2):93-104.
Group: nz.ac.waikato.cms.weka Artifact: localOutlierFactor
Show all versions Show documentation Show source
Show all versions Show documentation Show source
0 downloads
Artifact localOutlierFactor
Group nz.ac.waikato.cms.weka
Version 1.0.4
Last update 23. July 2013
Organization University of Waikato, Hamilton, NZ
URL http://weka.sourceforge.net/doc.packages/localOutlierFactor
License GNU General Public License 3
Dependencies amount 1
Dependencies weka-dev,
There are maybe transitive dependencies!
Group nz.ac.waikato.cms.weka
Version 1.0.4
Last update 23. July 2013
Organization University of Waikato, Hamilton, NZ
URL http://weka.sourceforge.net/doc.packages/localOutlierFactor
License GNU General Public License 3
Dependencies amount 1
Dependencies weka-dev,
There are maybe transitive dependencies!
oz-generic-levenshtein from group de.linuxusers.levenshtein (version 0.4.0)
YET ANOTHER IMPLEMENTATION OF LEVENSHTEIN DISTANCE
GenericLevenshtein is an implementation of Minimum Edit Distance, also called Levenshtein Distance, written by Ramon Ziai and Niels Ott. This algorithm is very popular and it is often used to compute the similarity of strings. The difference in the presented implementation is that it can operate on sequences of any Java object implementing equals(Object). So no matter if you want to compare genome sequences or sequences of numbers, or just strings, here you go!
Furthermore, the costs of the replace, insert, and delete operations can be customized by implementing the simple WeightCalculator<T> interface. In that case it is not a requirement to rely on equals(Object) as your implementation can do whatever you like it to do in oder to compare objects.
0 downloads
Artifact oz-generic-levenshtein
Group de.linuxusers.levenshtein
Version 0.4.0
Last update 11. September 2012
Organization not specified
URL http://niels.drni.de/s9y/pages/generic-levenshtein.html
License Apache License 2.0
Dependencies amount 0
Dependencies No dependencies
There are maybe transitive dependencies!
Group de.linuxusers.levenshtein
Version 0.4.0
Last update 11. September 2012
Organization not specified
URL http://niels.drni.de/s9y/pages/generic-levenshtein.html
License Apache License 2.0
Dependencies amount 0
Dependencies No dependencies
There are maybe transitive dependencies!
averagedOneDependenceEstimators from group nz.ac.waikato.cms.weka (version 1.2.1)
AODE achieves highly accurate classification by averaging over all of a small space of alternative naive-Bayes-like models that have weaker (and hence less detrimental) independence assumptions than naive Bayes. The resulting algorithm is computationally efficient while delivering highly accurate classification on many learning tasks. For more information, see G. Webb, J. Boughton, Z. Wang (2005). Not So Naive Bayes: Aggregating One-Dependence Estimators. Machine Learning. 58(1):5-24.
Group: nz.ac.waikato.cms.weka Artifact: averagedOneDependenceEstimators
Show all versions Show documentation Show source
Show all versions Show documentation Show source
0 downloads
Artifact averagedOneDependenceEstimators
Group nz.ac.waikato.cms.weka
Version 1.2.1
Last update 20. July 2012
Organization University of Waikato, Hamilton, NZ
URL http://weka.sourceforge.net/doc.packages/averagedOneDependenceEstimators
License GNU General Public License 3
Dependencies amount 1
Dependencies weka-dev,
There are maybe transitive dependencies!
Group nz.ac.waikato.cms.weka
Version 1.2.1
Last update 20. July 2012
Organization University of Waikato, Hamilton, NZ
URL http://weka.sourceforge.net/doc.packages/averagedOneDependenceEstimators
License GNU General Public License 3
Dependencies amount 1
Dependencies weka-dev,
There are maybe transitive dependencies!
sequentialInformationalBottleneckClusterer from group nz.ac.waikato.cms.weka (version 1.0.2)
Cluster data using the sequential information bottleneck algorithm.
Note: only hard clustering scheme is supported. sIB assign for each instance the cluster that have the minimum cost/distance to the instance. The trade-off beta is set to infinite so 1/beta is zero.
For more information, see:
Noam Slonim, Nir Friedman, Naftali Tishby: Unsupervised document classification using sequential information maximization. In: Proceedings of the 25th International ACM SIGIR Conference on Research and Development in Information Retrieval, 129-136, 2002.
Group: nz.ac.waikato.cms.weka Artifact: sequentialInformationalBottleneckClusterer
Show all versions Show documentation Show source
Show all versions Show documentation Show source
0 downloads
Artifact sequentialInformationalBottleneckClusterer
Group nz.ac.waikato.cms.weka
Version 1.0.2
Last update 26. April 2012
Organization University of Waikato, Hamilton, NZ
URL http://weka.sourceforge.net/doc.packages/sequentialInformationalBottleneckClusterer
License GNU General Public License 3
Dependencies amount 1
Dependencies weka-dev,
There are maybe transitive dependencies!
Group nz.ac.waikato.cms.weka
Version 1.0.2
Last update 26. April 2012
Organization University of Waikato, Hamilton, NZ
URL http://weka.sourceforge.net/doc.packages/sequentialInformationalBottleneckClusterer
License GNU General Public License 3
Dependencies amount 1
Dependencies weka-dev,
There are maybe transitive dependencies!
multiBoostAB from group nz.ac.waikato.cms.weka (version 1.0.2)
Class for boosting a classifier using the MultiBoosting method.
MultiBoosting is an extension to the highly successful AdaBoost technique for forming decision committees. MultiBoosting can be viewed as combining AdaBoost with wagging. It is able to harness both AdaBoost's high bias and variance reduction with wagging's superior variance reduction. Using C4.5 as the base learning algorithm, Multi-boosting is demonstrated to produce decision committees with lower error than either AdaBoost or wagging significantly more often than the reverse over a large representative cross-section of UCI data sets. It offers the further advantage over AdaBoost of suiting parallel execution.
For more information, see
Geoffrey I. Webb (2000). MultiBoosting: A Technique for Combining Boosting and Wagging. Machine Learning. Vol.40(No.2).
Group: nz.ac.waikato.cms.weka Artifact: multiBoostAB
Show all versions Show documentation Show source
Show all versions Show documentation Show source
0 downloads
Artifact multiBoostAB
Group nz.ac.waikato.cms.weka
Version 1.0.2
Last update 26. April 2012
Organization University of Waikato, Hamilton, NZ
URL http://weka.sourceforge.net/doc.packages/multiBoostAB
License GNU General Public License 3
Dependencies amount 1
Dependencies weka-dev,
There are maybe transitive dependencies!
Group nz.ac.waikato.cms.weka
Version 1.0.2
Last update 26. April 2012
Organization University of Waikato, Hamilton, NZ
URL http://weka.sourceforge.net/doc.packages/multiBoostAB
License GNU General Public License 3
Dependencies amount 1
Dependencies weka-dev,
There are maybe transitive dependencies!
- 1
- 2
- 3
- 4
- 5
- 6
- 7
- 8
- 9
- 10
- 11
- 12
- 13
- 14
- 15
- 16
- 17
- 18
- 19
- 20
- 21
- 22
- 23
- 24
- 25
- 26
- 27
- 28
- 29
- 30
- 31
- 32
- 33
- 34
- 35
- 36
- 37
- 38
- 39
- 40
- 41
- 42
- 43
- 44
- 45
- 46
- 47
- 48
- 49
- 50
- 51
- 52
- 53
- 54
- 55
- 56
- 57
- 58
- 59
- 60
- 61
- 62
- 63
- 64
- 65
- 66
- 67
- 68
- 69
- 70
- 71
- 72
- 73
- 74
- 75
- 76
- 77
- 78
- 79
- 80
- 81
- 82
- 83
- 84
- 85
- 86
- 87
- 88
- 89
- 90
- 91
- 92
- 93
- 94
- 95
- 96
- 97
- 98
- 99
- 100
- 101
- 102
Page 100 from 102 (items total 1015)
© 2015 - 2025 Weber Informatics LLC | Privacy Policy