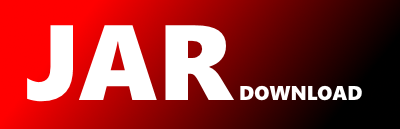
Download JAR files tagged by bagging with all dependencies
spark-bagging_2.11 from group com.github.pierrenodet (version 0.0.1)
0 downloads
rotationForest from group nz.ac.waikato.cms.weka (version 1.0.3)
Group: nz.ac.waikato.cms.weka Artifact: rotationForest
Show all versions Show documentation Show source
Show all versions Show documentation Show source
0 downloads
metaCost from group nz.ac.waikato.cms.weka (version 1.0.3)
This metaclassifier makes its base classifier cost-sensitive using the method specified in
Pedro Domingos: MetaCost: A general method for making classifiers cost-sensitive. In: Fifth International Conference on Knowledge Discovery and Data Mining, 155-164, 1999.
This classifier should produce similar results to one created by passing the base learner to Bagging, which is in turn passed to a CostSensitiveClassifier operating on minimum expected cost. The difference is that MetaCost produces a single cost-sensitive classifier of the base learner, giving the benefits of fast classification and interpretable output (if the base learner itself is interpretable). This implementation uses all bagging iterations when reclassifying training data (the MetaCost paper reports a marginal improvement when only those iterations containing each training instance are used in reclassifying that instance).
0 downloads
Artifact metaCost
Group nz.ac.waikato.cms.weka
Version 1.0.3
Last update 06. February 2013
Organization University of Waikato, Hamilton, NZ
URL http://weka.sourceforge.net/doc.packages/metaCost
License GNU General Public License 3
Dependencies amount 1
Dependencies weka-dev,
There are maybe transitive dependencies!
Group nz.ac.waikato.cms.weka
Version 1.0.3
Last update 06. February 2013
Organization University of Waikato, Hamilton, NZ
URL http://weka.sourceforge.net/doc.packages/metaCost
License GNU General Public License 3
Dependencies amount 1
Dependencies weka-dev,
There are maybe transitive dependencies!
decorate from group nz.ac.waikato.cms.weka (version 1.0.3)
DECORATE is a meta-learner for building diverse ensembles of classifiers by using specially constructed artificial training examples. Comprehensive experiments have demonstrated that this technique is consistently more accurate than the base classifier, Bagging and Random Forests. Decorate also obtains higher accuracy than Boosting on small training sets, and achieves comparable performance on larger training sets. For more details see: P. Melville, R. J. Mooney: Constructing Diverse Classifier Ensembles Using Artificial Training Examples. In: Eighteenth International Joint Conference on Artificial Intelligence, 505-510, 2003; P. Melville, R. J. Mooney (2004). Creating Diversity in Ensembles Using Artificial Data. Information Fusion: Special Issue on Diversity in Multiclassifier Systems.
1 downloads
Artifact decorate
Group nz.ac.waikato.cms.weka
Version 1.0.3
Last update 26. April 2012
Organization University of Waikato, Hamilton, NZ
URL http://weka.sourceforge.net/doc.packages/decorate
License GNU General Public License 3
Dependencies amount 2
Dependencies weka-dev, weka-dev,
There are maybe transitive dependencies!
Group nz.ac.waikato.cms.weka
Version 1.0.3
Last update 26. April 2012
Organization University of Waikato, Hamilton, NZ
URL http://weka.sourceforge.net/doc.packages/decorate
License GNU General Public License 3
Dependencies amount 2
Dependencies weka-dev, weka-dev,
There are maybe transitive dependencies!
Page 1 from 1 (items total 4)
© 2015 - 2024 Weber Informatics LLC | Privacy Policy