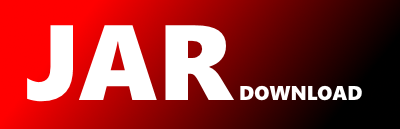
Download JAR files tagged by labels with all dependencies
mrglvq from group de.cit-ec.ml (version 0.1.0)
This project contains a Java implementation of median relational generalized learning vector
quantization as proposed by Nebel, Hammer, Frohberg, and Villmann
(2015, doi:10.1016/j.neucom.2014.12.096). Given a matrix of pairwise distances D and a
vector of labels Y it identifies prototypical data points (i.e. rows of D) which help
to classify the data set using a simple nearest neighbor rule. In particular, the algorithm
optimizes the generalized learning vector quantization cost function (Sato and Yamada, 1995)
via an expectation maximization scheme where in each iteration one prototype 'jumps' to
another data point in order to improve the cost function. If the cost function can not be
improved anymore for any of the data points, the algorithm terminates.
Artifact mrglvq
Group de.cit-ec.ml
Version 0.1.0
Last update 27. January 2018
Organization not specified
URL https://gitlab.ub.uni-bielefeld.de/bpaassen/median_relational_glvq
License The GNU General Public License, Version 3
Dependencies amount 1
Dependencies rng,
There are maybe transitive dependencies!
Group de.cit-ec.ml
Version 0.1.0
Last update 27. January 2018
Organization not specified
URL https://gitlab.ub.uni-bielefeld.de/bpaassen/median_relational_glvq
License The GNU General Public License, Version 3
Dependencies amount 1
Dependencies rng,
There are maybe transitive dependencies!
classificationViaClustering from group nz.ac.waikato.cms.weka (version 1.0.7)
A simple meta-classifier that uses a clusterer for classification. For cluster algorithms that use a fixed number of clusterers, like SimpleKMeans, the user has to make sure that the number of clusters to generate are the same as the number of class labels in the dataset in order to obtain a useful model.
Note: at prediction time, a missing value is returned if no cluster is found for the instance.
The code is based on the 'clusters to classes' functionality of the weka.clusterers.ClusterEvaluation class by Mark Hall.
Group: nz.ac.waikato.cms.weka Artifact: classificationViaClustering
Show all versions Show documentation Show source
Show all versions Show documentation Show source
2 downloads
Artifact classificationViaClustering
Group nz.ac.waikato.cms.weka
Version 1.0.7
Last update 26. November 2017
Organization University of Waikato, Hamilton, NZ
URL http://weka.sourceforge.net/doc.packages/classificationViaClustering
License GNU General Public License 3
Dependencies amount 1
Dependencies weka-dev,
There are maybe transitive dependencies!
Group nz.ac.waikato.cms.weka
Version 1.0.7
Last update 26. November 2017
Organization University of Waikato, Hamilton, NZ
URL http://weka.sourceforge.net/doc.packages/classificationViaClustering
License GNU General Public License 3
Dependencies amount 1
Dependencies weka-dev,
There are maybe transitive dependencies!
conjunctiveRule from group nz.ac.waikato.cms.weka (version 1.0.4)
This class implements a single conjunctive rule learner that can predict for numeric and nominal class labels.
A rule consists of antecedents "AND"ed together and the consequent (class value) for the classification/regression. In this case, the consequent is the distribution of the available classes (or mean for a numeric value) in the dataset. If the test instance is not covered by this rule, then it's predicted using the default class distributions/value of the data not covered by the rule in the training data.This learner selects an antecedent by computing the Information Gain of each antecendent and prunes the generated rule using Reduced Error Prunning (REP) or simple pre-pruning based on the number of antecedents.
For classification, the Information of one antecedent is the weighted average of the entropies of both the data covered and not covered by the rule.
For regression, the Information is the weighted average of the mean-squared errors of both the data covered and not covered by the rule.
In pruning, weighted average of the accuracy rates on the pruning data is used for classification while the weighted average of the mean-squared errors on the pruning data is used for regression.
Group: nz.ac.waikato.cms.weka Artifact: conjunctiveRule
Show all versions Show documentation Show source
Show all versions Show documentation Show source
0 downloads
Artifact conjunctiveRule
Group nz.ac.waikato.cms.weka
Version 1.0.4
Last update 29. April 2014
Organization University of Waikato, Hamilton, NZ
URL http://weka.sourceforge.net/doc.packages/conjunctiveRule
License GNU General Public License 3
Dependencies amount 1
Dependencies weka-dev,
There are maybe transitive dependencies!
Group nz.ac.waikato.cms.weka
Version 1.0.4
Last update 29. April 2014
Organization University of Waikato, Hamilton, NZ
URL http://weka.sourceforge.net/doc.packages/conjunctiveRule
License GNU General Public License 3
Dependencies amount 1
Dependencies weka-dev,
There are maybe transitive dependencies!
Page 8 from 8 (items total 73)
© 2015 - 2025 Weber Informatics LLC | Privacy Policy