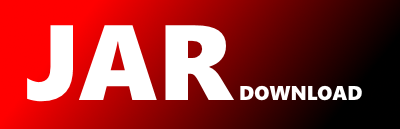
Download nz.ac.waikato.cms.weka.thirdparty JAR files with all dependencies
realAdaBoost from group nz.ac.waikato.cms.weka (version 1.0.2)
Class for boosting a 2-class classifier using the Real Adaboost method. For more information, see
J. Friedman, T. Hastie, R. Tibshirani (2000). Additive Logistic Regression: a Statistical View of Boosting. Annals of Statistics. 95(2):337-407.
scatterPlot3D from group nz.ac.waikato.cms.weka (version 1.0.4)
A visualization component for displaying a 3D scatter plot of the data using Java 3D. Requires Java 3D to be installed. This version adds built-in sampling controls to the GUI. The default sampling percentage is set so that a maximum of 5000 instances are plotted. The user can adjust this higher or lower to suit their available processing speed and memory.
1 downloads
isolationForest from group nz.ac.waikato.cms.weka (version 1.0.2)
Class for building and using a classifier built on the Isolation Forest anomaly detection algorithm. For more information see Fei Tony Liu, Kai Ming Ting and Zhi-Hua Zhou. 2008. Proceedings of the 2008 Eighth IEEE International Conference on Data Mining, pages 413-422.
partialLeastSquares from group nz.ac.waikato.cms.weka (version 1.0.4)
This package contains a filter for computing partial least squares and transforming the input data into the PLS space. It also contains a classifier for performing PLS regression.
1 downloads
mof from group org.andromda.thirdparty.jmi (version 20030918)
External (non-maven based) Andromda dependency.
jmiuml from group org.andromda.thirdparty.jmi (version 1.4di)
External (non-maven based) Andromda dependency.
zendesk-java-client from group com.cloudbees.thirdparty (version 0.0.2)
Java client for the Zendesk API
Group: com.cloudbees.thirdparty Artifact: zendesk-java-client
There is no JAR file uploaded. A download is not possible! Please choose another version.
1 downloads
gridSearch from group nz.ac.waikato.cms.weka (version 1.0.12)
Performs a grid search of parameter pairs for the a classifier (Y-axis, default is LinearRegression with the "Ridge" parameter) and the PLSFilter (X-axis, "# of Components") and chooses the best pair found for the actual predicting.
The initial grid is worked on with 2-fold CV to determine the values of the parameter pairs for the selected type of evaluation (e.g., accuracy). The best point in the grid is then taken and a 10-fold CV is performed with the adjacent parameter pairs. If a better pair is found, then this will act as new center and another 10-fold CV will be performed (kind of hill-climbing). This process is repeated until no better pair is found or the best pair is on the border of the grid.
In case the best pair is on the border, one can let GridSearch automatically extend the grid and continue the search. Check out the properties 'gridIsExtendable' (option '-extend-grid') and 'maxGridExtensions' (option '-max-grid-extensions <num>').
GridSearch can handle doubles, integers (values are just cast to int) and booleans (0 is false, otherwise true). float, char and long are supported as well.
The best filter/classifier setup can be accessed after the buildClassifier call via the getBestFilter/getBestClassifier methods.
Note on the implementation: after the data has been passed through the filter, a default NumericCleaner filter is applied to the data in order to avoid numbers that are getting too small and might produce NaNs in other schemes.
supervisedAttributeScaling from group nz.ac.waikato.cms.weka (version 1.0.2)
Package containing a class that rescales the attributes in a classification problem based on their discriminative power. This is useful as a pre-processing step for learning algorithms such as the k-nearest-neighbour method, to replace simple normalization. Each attribute is rescaled by multiplying it with a learned weight. All attributes excluding the class are assumed to be numeric and missing values are not permitted. To achieve the rescaling, this package also contains an implementation of non-negative logistic regression, which produces a logistic regression model with non-negative weights .
1 downloads
multiInstanceFilters from group nz.ac.waikato.cms.weka (version 1.0.10)
A collection of filters for manipulating multi-instance data. Includes PropositionalToMultiInstance, MultiInstanceToPropositional, MILESFilter and RELAGGS. For more information see:
M.-A. Krogel, S. Wrobel: Facets of Aggregation Approaches to Propositionalization. In: Work-in-Progress Track at the Thirteenth International Conference on Inductive Logic Programming (ILP), 2003.
Y. Chen, J. Bi, J.Z. Wang (2006). MILES: Multiple-instance learning via embedded instance selection. IEEE PAMI. 28(12):1931-1947.
James Foulds, Eibe Frank: Revisiting multiple-instance learning via embedded instance selection. In: 21st Australasian Joint Conference on Artificial Intelligence, 300-310, 2008.
1 downloads
libvirt-local-client from group net.java.dev.vcc.thirdparty (version 0.0.4)
This is an implementation of the Java binding of the libvirt api which binds to the
local libvirt library.
oneClassClassifier from group nz.ac.waikato.cms.weka (version 1.0.3)
Performs one-class classification on a dataset.
Classifier reduces the class being classified to just a single class, and learns the datawithout using any information from other classes. The testing stage will classify as 'target'or 'outlier' - so in order to calculate the outlier pass rate the dataset must contain informationfrom more than one class.
Also, the output varies depending on whether the label 'outlier' exists in the instances usedto build the classifier. If so, then 'outlier' will be predicted, if not, then the label willbe considered missing when the prediction does not favour the target class. The 'outlier' classwill not be used to build the model if there are instances of this class in the dataset. It cansimply be used as a flag, you do not need to relabel any classes.
For more information, see:
Kathryn Hempstalk, Eibe Frank, Ian H. Witten: One-Class Classification by Combining Density and Class Probability Estimation. In: Proceedings of the 12th European Conference on Principles and Practice of Knowledge Discovery in Databases and 19th European Conference on Machine Learning, ECMLPKDD2008, Berlin, 505--519, 2008.
visual from group com.googlecode.sarasvati.thirdparty.netbeans (version 7.3)
NetBeans Visual API
1 downloads
spring-data-batis from group com.minlia.cloud.thirdparty (version 1.0.0.RELEASE)
The primary goal of the Spring Data project is to make it easier to build
Spring-powered applications
that use data access technologies. This module deals with enhanced support for MyBatis based
data access layers.
1 downloads
SPegasos from group nz.ac.waikato.cms.weka (version 1.0.2)
Implements the stochastic variant of the Pegasos (Primal Estimated sub-GrAdient SOlver for SVM) method of Shalev-Shwartz et al. (2007). This implementation globally replaces all missing values and transforms nominal attributes into binary ones. It also normalizes all attributes, so the coefficients in the output are based on the normalized data. Can either minimize the hinge loss (SVM) or log loss (logistic regression). For more information, see
S. Shalev-Shwartz, Y. Singer, N. Srebro: Pegasos: Primal Estimated sub-GrAdient SOlver for SVM. In: 24th International Conference on MachineLearning, 807-814, 2007.
Group: nz.ac.waikato.cms.weka Artifact: SPegasos
There is no JAR file uploaded. A download is not possible! Please choose another version.
1 downloads
Page 11 from 23 (items total 338)
© 2015 - 2025 Weber Informatics LLC | Privacy Policy