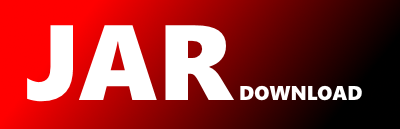
Download JAR files tagged by conference with all dependencies
sequentialInformationalBottleneckClusterer from group nz.ac.waikato.cms.weka (version 1.0.2)
Cluster data using the sequential information bottleneck algorithm.
Note: only hard clustering scheme is supported. sIB assign for each instance the cluster that have the minimum cost/distance to the instance. The trade-off beta is set to infinite so 1/beta is zero.
For more information, see:
Noam Slonim, Nir Friedman, Naftali Tishby: Unsupervised document classification using sequential information maximization. In: Proceedings of the 25th International ACM SIGIR Conference on Research and Development in Information Retrieval, 129-136, 2002.
Group: nz.ac.waikato.cms.weka Artifact: sequentialInformationalBottleneckClusterer
Show all versions Show documentation Show source
Show all versions Show documentation Show source
0 downloads
Artifact sequentialInformationalBottleneckClusterer
Group nz.ac.waikato.cms.weka
Version 1.0.2
Last update 26. April 2012
Organization University of Waikato, Hamilton, NZ
URL http://weka.sourceforge.net/doc.packages/sequentialInformationalBottleneckClusterer
License GNU General Public License 3
Dependencies amount 1
Dependencies weka-dev,
There are maybe transitive dependencies!
Group nz.ac.waikato.cms.weka
Version 1.0.2
Last update 26. April 2012
Organization University of Waikato, Hamilton, NZ
URL http://weka.sourceforge.net/doc.packages/sequentialInformationalBottleneckClusterer
License GNU General Public License 3
Dependencies amount 1
Dependencies weka-dev,
There are maybe transitive dependencies!
SPegasos from group nz.ac.waikato.cms.weka (version 1.0.2)
Implements the stochastic variant of the Pegasos (Primal Estimated sub-GrAdient SOlver for SVM) method of Shalev-Shwartz et al. (2007). This implementation globally replaces all missing values and transforms nominal attributes into binary ones. It also normalizes all attributes, so the coefficients in the output are based on the normalized data. Can either minimize the hinge loss (SVM) or log loss (logistic regression). For more information, see
S. Shalev-Shwartz, Y. Singer, N. Srebro: Pegasos: Primal Estimated sub-GrAdient SOlver for SVM. In: 24th International Conference on MachineLearning, 807-814, 2007.
1 downloads
Artifact SPegasos
Group nz.ac.waikato.cms.weka
Version 1.0.2
Last update 26. April 2012
Organization University of Waikato, Hamilton, NZ
URL http://weka.sourceforge.net/doc.packages/SPegasos
License GNU General Public License 3
Dependencies amount 1
Dependencies weka-dev,
There are maybe transitive dependencies!
Group nz.ac.waikato.cms.weka
Version 1.0.2
Last update 26. April 2012
Organization University of Waikato, Hamilton, NZ
URL http://weka.sourceforge.net/doc.packages/SPegasos
License GNU General Public License 3
Dependencies amount 1
Dependencies weka-dev,
There are maybe transitive dependencies!
decorate from group nz.ac.waikato.cms.weka (version 1.0.3)
DECORATE is a meta-learner for building diverse ensembles of classifiers by using specially constructed artificial training examples. Comprehensive experiments have demonstrated that this technique is consistently more accurate than the base classifier, Bagging and Random Forests. Decorate also obtains higher accuracy than Boosting on small training sets, and achieves comparable performance on larger training sets. For more details see: P. Melville, R. J. Mooney: Constructing Diverse Classifier Ensembles Using Artificial Training Examples. In: Eighteenth International Joint Conference on Artificial Intelligence, 505-510, 2003; P. Melville, R. J. Mooney (2004). Creating Diversity in Ensembles Using Artificial Data. Information Fusion: Special Issue on Diversity in Multiclassifier Systems.
1 downloads
Artifact decorate
Group nz.ac.waikato.cms.weka
Version 1.0.3
Last update 26. April 2012
Organization University of Waikato, Hamilton, NZ
URL http://weka.sourceforge.net/doc.packages/decorate
License GNU General Public License 3
Dependencies amount 2
Dependencies weka-dev, weka-dev,
There are maybe transitive dependencies!
Group nz.ac.waikato.cms.weka
Version 1.0.3
Last update 26. April 2012
Organization University of Waikato, Hamilton, NZ
URL http://weka.sourceforge.net/doc.packages/decorate
License GNU General Public License 3
Dependencies amount 2
Dependencies weka-dev, weka-dev,
There are maybe transitive dependencies!
oneClassClassifier from group nz.ac.waikato.cms.weka (version 1.0.4)
Performs one-class classification on a dataset.
Classifier reduces the class being classified to just a single class, and learns the datawithout using any information from other classes. The testing stage will classify as 'target'or 'outlier' - so in order to calculate the outlier pass rate the dataset must contain informationfrom more than one class.
Also, the output varies depending on whether the label 'outlier' exists in the instances usedto build the classifier. If so, then 'outlier' will be predicted, if not, then the label willbe considered missing when the prediction does not favour the target class. The 'outlier' classwill not be used to build the model if there are instances of this class in the dataset. It cansimply be used as a flag, you do not need to relabel any classes.
For more information, see:
Kathryn Hempstalk, Eibe Frank, Ian H. Witten: One-Class Classification by Combining Density and Class Probability Estimation. In: Proceedings of the 12th European Conference on Principles and Practice of Knowledge Discovery in Databases and 19th European Conference on Machine Learning, ECMLPKDD2008, Berlin, 505--519, 2008.
Group: nz.ac.waikato.cms.weka Artifact: oneClassClassifier
Show all versions Show documentation Show source
Show all versions Show documentation Show source
3 downloads
Artifact oneClassClassifier
Group nz.ac.waikato.cms.weka
Version 1.0.4
Last update 14. May 2013
Organization University of Waikato, Hamilton, NZ
URL http://weka.sourceforge.net/doc.packages/oneClassClassifier
License GNU General Public License 3
Dependencies amount 1
Dependencies weka-dev,
There are maybe transitive dependencies!
Group nz.ac.waikato.cms.weka
Version 1.0.4
Last update 14. May 2013
Organization University of Waikato, Hamilton, NZ
URL http://weka.sourceforge.net/doc.packages/oneClassClassifier
License GNU General Public License 3
Dependencies amount 1
Dependencies weka-dev,
There are maybe transitive dependencies!
paceRegression from group nz.ac.waikato.cms.weka (version 1.0.2)
Class for building pace regression linear models and using them for prediction.
Under regularity conditions, pace regression is provably optimal when the number of coefficients tends to infinity. It consists of a group of estimators that are either overall optimal or optimal under certain conditions.
The current work of the pace regression theory, and therefore also this implementation, do not handle:
- missing values
- non-binary nominal attributes
- the case that n - k is small where n is the number of instances and k is the number of coefficients (the threshold used in this implmentation is 20)
For more information see:
Wang, Y (2000). A new approach to fitting linear models in high dimensional spaces. Hamilton, New Zealand.
Wang, Y., Witten, I. H.: Modeling for optimal probability prediction. In: Proceedings of the Nineteenth International Conference in Machine Learning, Sydney, Australia, 650-657, 2002.
Group: nz.ac.waikato.cms.weka Artifact: paceRegression
Show all versions Show documentation Show source
Show all versions Show documentation Show source
0 downloads
Artifact paceRegression
Group nz.ac.waikato.cms.weka
Version 1.0.2
Last update 26. April 2012
Organization University of Waikato, Hamilton, NZ
URL http://weka.sourceforge.net/doc.packages/paceRegression
License GNU General Public License 3
Dependencies amount 1
Dependencies weka-dev,
There are maybe transitive dependencies!
Group nz.ac.waikato.cms.weka
Version 1.0.2
Last update 26. April 2012
Organization University of Waikato, Hamilton, NZ
URL http://weka.sourceforge.net/doc.packages/paceRegression
License GNU General Public License 3
Dependencies amount 1
Dependencies weka-dev,
There are maybe transitive dependencies!
boilerpipe from group de.l3s.boilerpipe (version 1.1.0)
The boilerpipe library provides algorithms to detect and remove the surplus "clutter" (boilerplate, templates) around the main textual content of a web page.
The library already provides specific strategies for common tasks (for example: news article extraction) and may also be easily extended for individual problem settings.
Extracting content is very fast (milliseconds), just needs the input document (no global or site-level information required) and is usually quite accurate.
Boilerpipe is a Java library written by Christian Kohlschütter. It is released under the Apache License 2.0.
The algorithms used by the library are based on (and extending) some concepts of the paper "Boilerplate Detection using Shallow Text Features" by Christian Kohlschütter et al., presented at WSDM 2010 -- The Third ACM International Conference on Web Search and Data Mining New York City, NY USA.
10 downloads
Artifact boilerpipe
Group de.l3s.boilerpipe
Version 1.1.0
Last update 03. November 2010
Organization not specified
URL http://code.google.com/p/boilerpipe/
License Apache License 2.0
Dependencies amount 0
Dependencies No dependencies
There are maybe transitive dependencies!
Group de.l3s.boilerpipe
Version 1.1.0
Last update 03. November 2010
Organization not specified
URL http://code.google.com/p/boilerpipe/
License Apache License 2.0
Dependencies amount 0
Dependencies No dependencies
There are maybe transitive dependencies!
Page 4 from 4 (items total 36)
© 2015 - 2025 Weber Informatics LLC | Privacy Policy